Method for quantitatively predicting cartilage repair rate after mesenchymal stem cell therapy through machine learning
A technology of machine learning and cartilage repair, applied in the field of regenerative medicine, can solve the problems of impossibility, high data, high time and cost, and small data (several to dozens, etc.), so as to improve the quality of prediction and prevent fluctuation and divergence , optimize the effect of the combination
- Summary
- Abstract
- Description
- Claims
- Application Information
AI Technical Summary
Problems solved by technology
Method used
Examples
Embodiment
[0035] The present invention provides a machine learning method for quantitatively predicting cartilage repair rate after mesenchymal stem cell therapy, which aims to develop a machine learning model by training a database generated from published clinical trial reports, and then use the Interrelationships and correlations among attributes to predict efficacy of MSC transplantation for cartilage repair. The present invention mainly includes several major processes of database establishment, preprocessing of original data, missing data processing, model development, evaluation of model accuracy, and generation of MSC treatment guideline for cartilage repair.
[0036] The above process will be described in detail below.
[0037] 1. Database establishment
[0038] Gather published clinical trial reports on MSC cartilage repair therapies (e.g. from PubMed and the International Cartilage Repair Society (ICRS) database for published reports including traumatic cartilage defects, os...
PUM
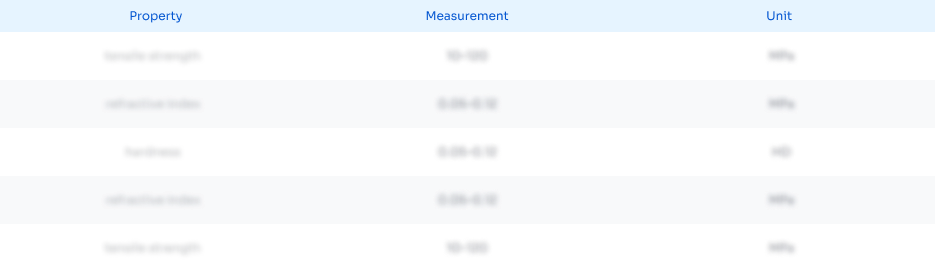
Abstract
Description
Claims
Application Information
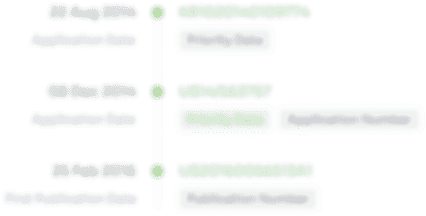
- R&D Engineer
- R&D Manager
- IP Professional
- Industry Leading Data Capabilities
- Powerful AI technology
- Patent DNA Extraction
Browse by: Latest US Patents, China's latest patents, Technical Efficacy Thesaurus, Application Domain, Technology Topic, Popular Technical Reports.
© 2024 PatSnap. All rights reserved.Legal|Privacy policy|Modern Slavery Act Transparency Statement|Sitemap|About US| Contact US: help@patsnap.com