Reservoir downstream water level prediction method based on deep learning model
A technology of deep learning and prediction methods, which is applied in neural learning methods, predictions, biological neural network models, etc., can solve problems such as time-consuming solutions, complex modeling, adverse effects of reservoir scheduling, etc., and achieve the effect of improving prediction accuracy
- Summary
- Abstract
- Description
- Claims
- Application Information
AI Technical Summary
Problems solved by technology
Method used
Image
Examples
Embodiment
[0087] Gezhouba present invention in the study, using the 2017 data to verify the validity and accuracy of the model of the present invention. The downstream water level for 12 months in 2017 as a validation set. For monthly validation set, using the first two months of data corresponding to a training set, as a period of 2 hours.
[0088] Select inflow historical periods (Q i ), The discharged volume (Q o ), The upstream water level (z u ), Head (H) and the downstream water level (z d ) As a possible impact factor, the downstream water level calculated maximum coefficient information (the MIC), as shown in Table 1. Table 1 lists an example in March and August of MIC values of the two sets of validation, table factor is greater than 0.6 using a gray fill. Wherein represents the current time t-0, t-1 represents a period of time before, and so on. Thus, March validation set related factor Q o,t ~ Q o,t-8 And Z d,t-1 ~ Z d,t-8 August correlation factor for the validation set Q i,t ...
PUM
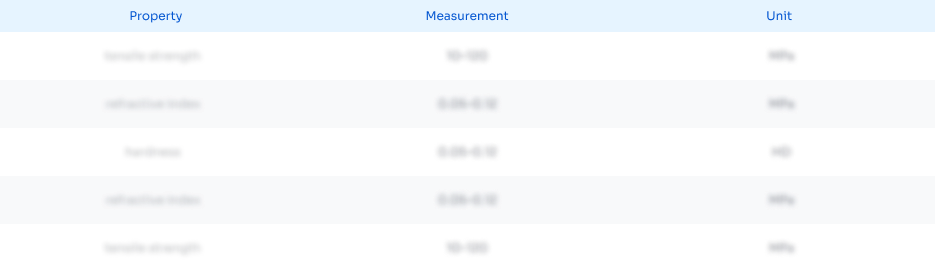
Abstract
Description
Claims
Application Information
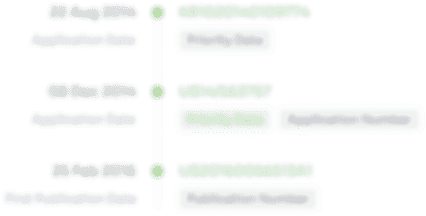
- R&D
- Intellectual Property
- Life Sciences
- Materials
- Tech Scout
- Unparalleled Data Quality
- Higher Quality Content
- 60% Fewer Hallucinations
Browse by: Latest US Patents, China's latest patents, Technical Efficacy Thesaurus, Application Domain, Technology Topic, Popular Technical Reports.
© 2025 PatSnap. All rights reserved.Legal|Privacy policy|Modern Slavery Act Transparency Statement|Sitemap|About US| Contact US: help@patsnap.com