Deep learning neural network cascade model-based human heart coronary artery extraction method
A neural network and cascade model technology, applied in biological neural network model, neural architecture, image data processing and other directions, can solve the problems of missing small blood vessels, unrecognized small blood vessels, low contrast and other problems in segmentation results
- Summary
- Abstract
- Description
- Claims
- Application Information
AI Technical Summary
Problems solved by technology
Method used
Image
Examples
Embodiment
[0034] Please refer to figure 1 As shown, the present invention discloses a human heart coronary artery extraction method based on a deep learning neural network cascade model, which mainly includes four steps of S1-S4.
[0035] S1. Preprocessing of the original image of the coronary CT sequence.
[0036] The CT sequence is stored in the Dicom file format, and the original image of the CT sequence is converted into a picture format according to a certain window width and window level to obtain the CT sequence picture. The image format adopted in this embodiment is jpg. The window width and level are dynamically adjusted to ensure that blood vessels with a diameter of more than 1.5 mm in the image can be clearly displayed. In this embodiment, the window width and level are 400 and 70.
[0037] S2. Segmentation of the whole image.
[0038] The CT sequence images are segmented by the pre-trained full-image model, and the segmentation results of the main coronary artery and the...
PUM
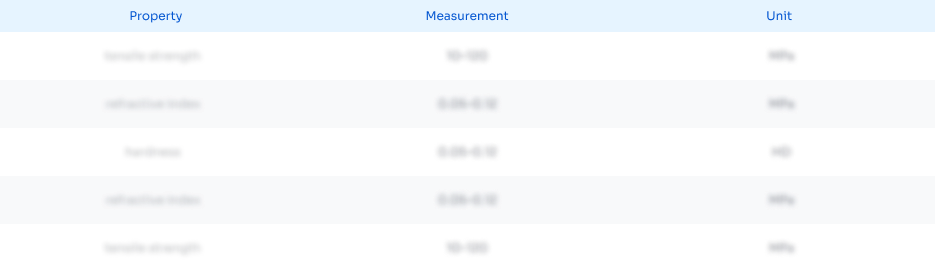
Abstract
Description
Claims
Application Information
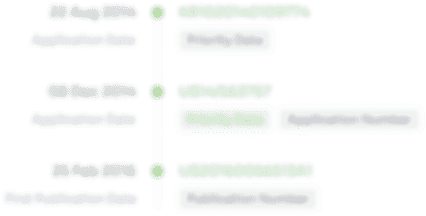
- R&D Engineer
- R&D Manager
- IP Professional
- Industry Leading Data Capabilities
- Powerful AI technology
- Patent DNA Extraction
Browse by: Latest US Patents, China's latest patents, Technical Efficacy Thesaurus, Application Domain, Technology Topic, Popular Technical Reports.
© 2024 PatSnap. All rights reserved.Legal|Privacy policy|Modern Slavery Act Transparency Statement|Sitemap|About US| Contact US: help@patsnap.com