Course field multi-modal document classification method based on cross-modal attention convolutional neural network
A convolutional neural network, multimodal technology, applied in the field of multimodal document classification in the course field based on cross-modal attention convolutional neural network, can solve difficult image and text semantic feature vectors, reduce multimodal document Accuracy of feature expression, hindering the performance of multimodal document classification tasks, etc.
- Summary
- Abstract
- Description
- Claims
- Application Information
AI Technical Summary
Problems solved by technology
Method used
Image
Examples
Embodiment Construction
[0074] Now in conjunction with embodiment, accompanying drawing, the present invention will be further described:
[0075] The multimodal document classification method in the curriculum field based on the cross-modal attention convolutional neural network proposed by the present invention is mainly divided into five modules: 1) preprocessing of multimodal document data. 2) Image feature construction of dense convolutional neural network based on attention mechanism. 3) Two-way long-short-term memory network text feature construction based on attention mechanism. 4) Group cross-modal fusion based on attention mechanism. 5) Multi-label classification of multi-modal documents. The model diagram of the whole method is shown in figure 1 shown, specifically as follows:
[0076] 1. Preprocessing of multimodal document data
[0077] Represent the mixed-text multimodal document as Represents the dth multimodal document data. in I d is the multimodal document data list that ...
PUM
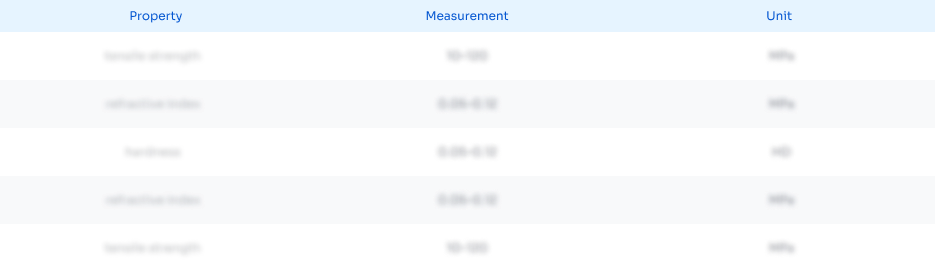
Abstract
Description
Claims
Application Information
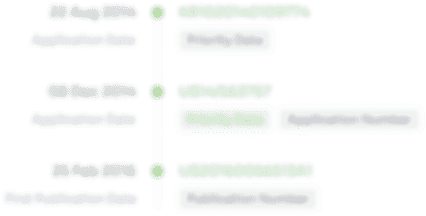
- R&D Engineer
- R&D Manager
- IP Professional
- Industry Leading Data Capabilities
- Powerful AI technology
- Patent DNA Extraction
Browse by: Latest US Patents, China's latest patents, Technical Efficacy Thesaurus, Application Domain, Technology Topic, Popular Technical Reports.
© 2024 PatSnap. All rights reserved.Legal|Privacy policy|Modern Slavery Act Transparency Statement|Sitemap|About US| Contact US: help@patsnap.com