Semi-automatic model updating system and model updating method
A model update and semi-automatic technology, applied in the field of Internet information security, can solve the problems of low data collection efficiency and accuracy, model update coverage and accuracy, etc., to improve the ability to identify abnormal data and strengthen the ability to learn and express , high accuracy and high efficiency
- Summary
- Abstract
- Description
- Claims
- Application Information
AI Technical Summary
Problems solved by technology
Method used
Image
Examples
Embodiment 1
[0044] see figure 1 As shown, a semi-automatic model update system includes a data preprocessing module, a suspicious data extraction module, a CNN model training module and a CNN model update module; the suspicious data extraction module includes a strategy marking module, a Cluster model marking module, and a manual marking module Module and Feature Visualization Markup Module.
[0045] Wherein, the data preprocessing module is used to perform standardized preprocessing on the original data to obtain a standardized data set, so as to improve the calculation efficiency of the data. Specifically include: (1) Determine the Schema of the data, and extract the main information from the userAgent, such as: device name, version, browser, version, etc. For example, the trajectory data is processed into a standard array, etc.; (2) the original row storage is converted into a column storage, reducing a large number of unnecessary repeated analysis; (3) pre-aggregation processing: suc...
Embodiment 2
[0063] see Figure 4 As shown, a semi-automatic model update system, compared with Embodiment 1, the difference is that the shown model update system also includes a suspicious data pattern division module, which is used to divide the pattern of the suspicious data set and eliminate Suspicious samples whose recognition degree of the original CNN model is lower than a preset threshold value are obtained to obtain several patterns of suspicious samples, so as to improve the recognition degree of suspicious samples in the original CNN model and reduce the risk of false sealing of the CNN model.
[0064] Further, the CNN model training module uses the suspicious samples of the several modes as training samples, and adopts the same method as that in Embodiment 1 to train the original CNN model to obtain a model training report and a new version of the CNN model.
Embodiment 3
[0066] see Figure 5 As shown, a semi-automatic model updating method adopts the semi-automatic model updating system described in Embodiment 1 to update the model, comprising the following steps:
[0067] S1. Create an original CNN model and deploy the original CNN model online;
[0068] S2. Perform standardized preprocessing on the original data to obtain a standardized data set;
[0069] S3. Regularly (7 days) extract and mark the suspicious data in the standardized data set through strategy marking, Cluster model marking, manual analysis marking and feature visualization marking of the original CNN model to obtain a suspicious data set;
[0070] S4. Using the suspicious data set as a training sample, train the original CNN model to obtain a model training report and a new version of the CNN model;
[0071] S5. According to the model training report, the data modeling engineer checks the recognition degree of the new version of CNN model for the suspicious samples and the f...
PUM
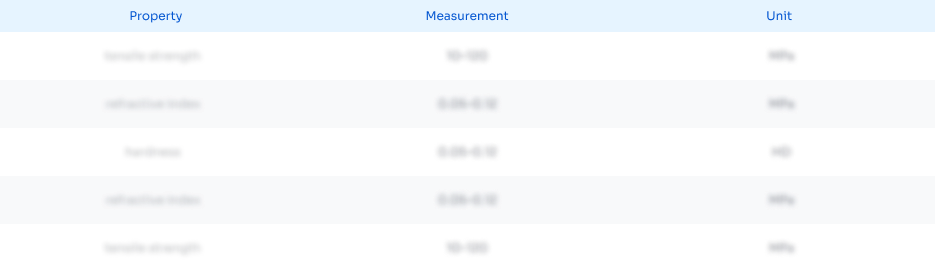
Abstract
Description
Claims
Application Information
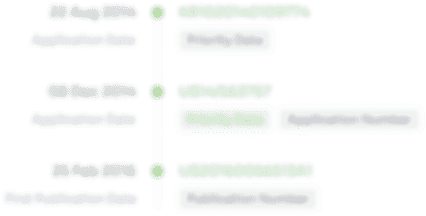
- R&D Engineer
- R&D Manager
- IP Professional
- Industry Leading Data Capabilities
- Powerful AI technology
- Patent DNA Extraction
Browse by: Latest US Patents, China's latest patents, Technical Efficacy Thesaurus, Application Domain, Technology Topic, Popular Technical Reports.
© 2024 PatSnap. All rights reserved.Legal|Privacy policy|Modern Slavery Act Transparency Statement|Sitemap|About US| Contact US: help@patsnap.com