Depth binary feature facial expression recognition method based on lightweight network
A facial expression recognition, lightweight technology, applied in the field of facial expression recognition
- Summary
- Abstract
- Description
- Claims
- Application Information
AI Technical Summary
Problems solved by technology
Method used
Image
Examples
Embodiment Construction
[0063] The technical solutions in the embodiments of the present invention will be described clearly and in detail below with reference to the drawings in the embodiments of the present invention. The described embodiments are only some of the embodiments of the invention.
[0064] The technical scheme that the present invention solves the problems of the technologies described above is:
[0065] The embodiment of the present invention is implemented based on a lightweight multi-layer binary-traditional composite convolutional neural network, where the traditional feature extraction task is performed before the network is executed, and the deep feature extraction is performed during network training, and the output of each layer of the network is traditional The fusion of features and deep features is used as the input of the next layer network.
[0066] Below in conjunction with accompanying drawing, the present invention will be further described:
[0067] as attached fig...
PUM
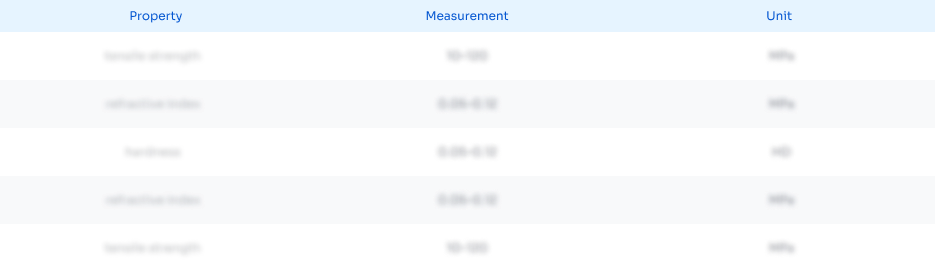
Abstract
Description
Claims
Application Information
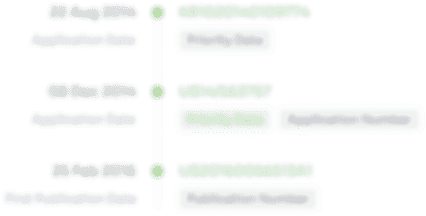
- R&D Engineer
- R&D Manager
- IP Professional
- Industry Leading Data Capabilities
- Powerful AI technology
- Patent DNA Extraction
Browse by: Latest US Patents, China's latest patents, Technical Efficacy Thesaurus, Application Domain, Technology Topic, Popular Technical Reports.
© 2024 PatSnap. All rights reserved.Legal|Privacy policy|Modern Slavery Act Transparency Statement|Sitemap|About US| Contact US: help@patsnap.com