Electronic component quality detection method and system based on deep learning
A quality inspection method and technology of electronic components, applied in neural learning methods, instruments, image analysis, etc., can solve the problems of loss of accuracy and inability to guarantee accuracy, improve accuracy and speed, reduce network overhead, and increase detection accuracy Effect
- Summary
- Abstract
- Description
- Claims
- Application Information
AI Technical Summary
Problems solved by technology
Method used
Image
Examples
Embodiment Construction
[0042] In order to make the features and advantages of this patent more obvious and easy to understand, the following specific examples are given and described in detail as follows: Example: an electronic component detection method based on an improved deep learning convolutional neural network, first collect unqualified electronic components. Component images, divide the images into training set, validation set and test set, and mark the unqualified areas of the images in the dataset; secondly build a convolutional neural network model for crack image detection; then use the images in the training dataset Train the convolutional neural network model for unqualified image detection; use the trained full convolutional neural network model for unqualified image detection to perform appearance detection on unqualified images in the test dataset.
[0043] Further, the main steps of using the images in the training data set to train the fully convolutional neural network model for q...
PUM
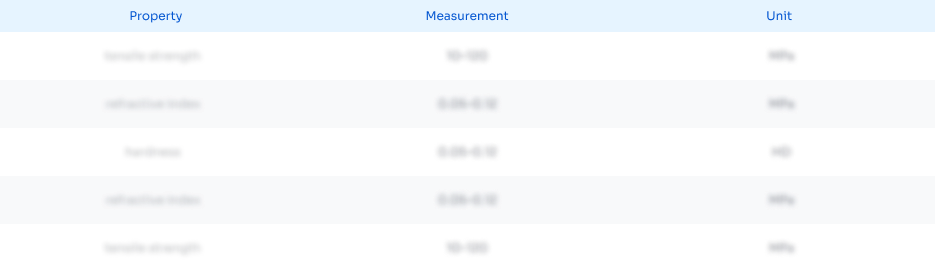
Abstract
Description
Claims
Application Information
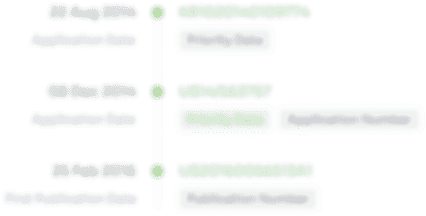
- R&D Engineer
- R&D Manager
- IP Professional
- Industry Leading Data Capabilities
- Powerful AI technology
- Patent DNA Extraction
Browse by: Latest US Patents, China's latest patents, Technical Efficacy Thesaurus, Application Domain, Technology Topic, Popular Technical Reports.
© 2024 PatSnap. All rights reserved.Legal|Privacy policy|Modern Slavery Act Transparency Statement|Sitemap|About US| Contact US: help@patsnap.com