Logging lithology recognition method based on convolutional neural network learning
A convolutional neural network and lithology identification technology, applied in neural learning methods, biological neural network models, neural architectures, etc., can solve problems such as slow convergence speed, gradient explosion, easy access to gradient disappearance, etc., to prevent overfitting The effects of sum and underfitting, comprehensive formation and lithology information, and improved applicability
- Summary
- Abstract
- Description
- Claims
- Application Information
AI Technical Summary
Problems solved by technology
Method used
Image
Examples
Embodiment Construction
[0026] In order to make the object, technical solution and advantages of the present invention clearer, the specific implementation manners of the present invention will be further described in detail below in conjunction with the accompanying drawings. Here, the exemplary embodiments and descriptions of the present invention are used to explain the present invention, but not to limit the present invention.
[0027] Examples are attached figure 1 The programming interface API provided by TensorFlow defines convolutional neural networks. TensorFlow is an open source software library developed by Google that uses data flow graphs for numerical calculations. Based on the above-mentioned source program, the process flow of the automatic identification method for logging lithology based on convolutional neural network machine learning designed by the present invention is shown in the appendix figure 1 , all steps can be automatically run by those skilled in the art using computer ...
PUM
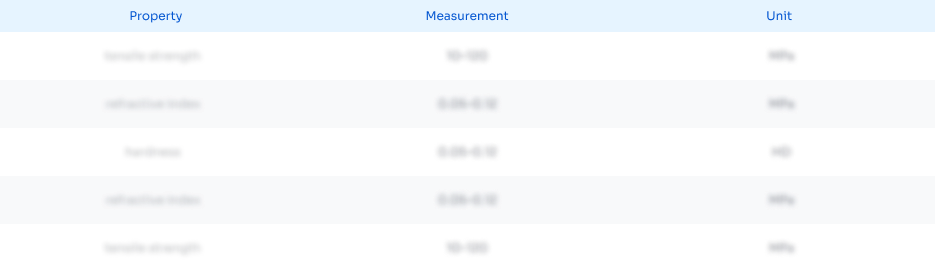
Abstract
Description
Claims
Application Information
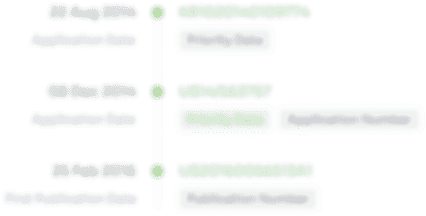
- R&D Engineer
- R&D Manager
- IP Professional
- Industry Leading Data Capabilities
- Powerful AI technology
- Patent DNA Extraction
Browse by: Latest US Patents, China's latest patents, Technical Efficacy Thesaurus, Application Domain, Technology Topic, Popular Technical Reports.
© 2024 PatSnap. All rights reserved.Legal|Privacy policy|Modern Slavery Act Transparency Statement|Sitemap|About US| Contact US: help@patsnap.com