Health state and residual life prediction method of multi-type lithium ion battery pack management system
A technology of lithium-ion battery packs and lithium-ion batteries, which is applied in the direction of measuring electricity, measuring devices, and measuring electrical variables, and can solve problems such as poor prediction accuracy and difficult battery balance management
- Summary
- Abstract
- Description
- Claims
- Application Information
AI Technical Summary
Problems solved by technology
Method used
Image
Examples
specific Embodiment approach 1
[0040] Specific embodiment one: figure 1 This embodiment is a simplified structural diagram of a multi-type lithium-ion battery pack management system in an actual application scenario. The system consists of multiple independent lithium-ion battery management systems, and each system can use different types of lithium-ion batteries. Each independent system is independently responsible for completing its own balance management, temperature warning and SOH prediction functions. Such a management structure can ensure that when a certain part fails, it will not affect the overall operation.
[0041] figure 2 Schematic diagram of the composition of the lithium-ion battery management system. Each management system consists of three modules (not including battery), which are the controller module, the sensor module, and the memory and information transmission module.
[0042] The controller module consists of an STM32 chip, a charge and discharge power controller, and a cooling fan. T...
specific Embodiment approach 2
[0051] Embodiment 2: This embodiment further explains the method for predicting health and remaining life based on the reconfigurable lithium-ion battery management system described in Embodiment 1. The steps of independent principal component analysis described in step 1 are as follows :
[0052] Record the capacity degradation data of the lithium-ion battery pack as: (Abbreviated as C), where n is the number of the single battery of the same type, and m is the number of cycles of the single battery.
[0053] Step 1. Subtract C from its mean
[0054] Step 2: Calculate R=EDE T , Where R is the covariance matrix of C, E is the orthogonal matrix of eigenvectors, and D is the diagonal matrix of its eigenvalues;
[0055] Step 3: Calculate the whitening data C v =ED -1 / 2 E T x;
[0056] Step 4: Set the number of iterations p, and initialize the vector W randomly p , So that the sum of each row is 1. Then according to the formula
[0057]
[0058] Find W at time i i . Where g'(.) is the s...
specific Embodiment approach 3
[0061] Specific embodiment three: This embodiment is to further explain the method for predicting the state of health and remaining life of the multi-type lithium-ion battery pack management system described in the first embodiment. Figure 4 It is a flow chart of establishing a multi-scale combination model of single battery in the present invention. The specific process of establishing a multi-scale combined model described in step 2 and step 3 to realize the prediction of lithium-ion pre-health state and remaining life is as follows:
[0062] Step 1. Use discrete wavelet decomposition to decompose the monomer capacity degradation data into two parts: high frequency fluctuation and low frequency trend;
[0063] Step 2: Bring the high-frequency fluctuation part and low-frequency trend part of the same battery (except the test data) obtained in step 1 into the residual wavelet neural network for training;
[0064] Step 3: Combine the residual wavelet network of the trained low-freque...
PUM
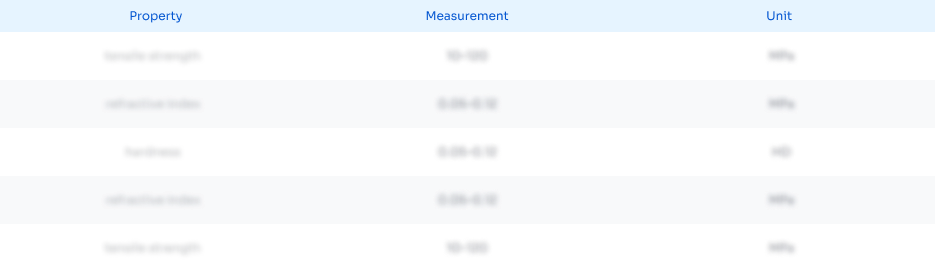
Abstract
Description
Claims
Application Information
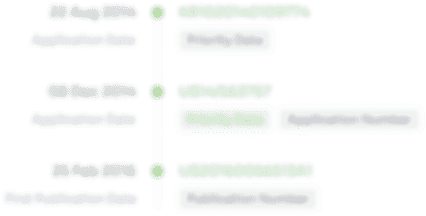
- R&D Engineer
- R&D Manager
- IP Professional
- Industry Leading Data Capabilities
- Powerful AI technology
- Patent DNA Extraction
Browse by: Latest US Patents, China's latest patents, Technical Efficacy Thesaurus, Application Domain, Technology Topic, Popular Technical Reports.
© 2024 PatSnap. All rights reserved.Legal|Privacy policy|Modern Slavery Act Transparency Statement|Sitemap|About US| Contact US: help@patsnap.com