Neural network model input channel integration method for transfer learning
A neural network model and input channel technology, which is applied in the field of neural network model input channel integration, can solve the problems of occupying memory space, increasing the calculation amount of neural network, and increasing the processing time of copy channel, so as to reduce the amount of calculation, reduce the pressure, shorten the The effect of processing time
- Summary
- Abstract
- Description
- Claims
- Application Information
AI Technical Summary
Problems solved by technology
Method used
Image
Examples
Embodiment Construction
[0038] The invention relates to a neural network model input channel integration method for migration learning, which is suitable for various general target detection and recognition algorithms and network frameworks based on convolutional neural networks, and realizes data exchange between heterogeneous training data and actual test data Uniform format.
[0039] A neural network model input channel integration method for transfer learning, the steps are as follows:
[0040] Step 1: Modify the data input layer description in the network model description file, delete the original data input layer description, and add the data input layer description based on the image data list; and rename the first convolutional layer to be modified;
[0041] The present invention is not limited to a specific neural network framework, and can be implemented for mainstream frameworks tensorflow, caffe, and pytorch. The following is a specific description, and the caffe framework is temporarily...
PUM
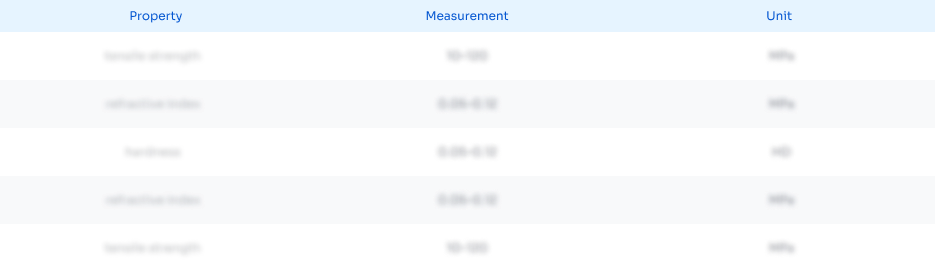
Abstract
Description
Claims
Application Information
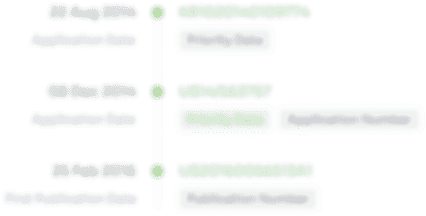
- R&D Engineer
- R&D Manager
- IP Professional
- Industry Leading Data Capabilities
- Powerful AI technology
- Patent DNA Extraction
Browse by: Latest US Patents, China's latest patents, Technical Efficacy Thesaurus, Application Domain, Technology Topic, Popular Technical Reports.
© 2024 PatSnap. All rights reserved.Legal|Privacy policy|Modern Slavery Act Transparency Statement|Sitemap|About US| Contact US: help@patsnap.com