Cross rod end fastening bolt loss fault detection method based on deep learning
An end tightening and deep learning technology, applied in computer parts, railway car body parts, railway vehicle testing, etc., can solve the problems of low detection efficiency, high labor cost and time cost, loss of fastening bolts, etc. The effect of improving fault detection speed, reducing labor cost and time cost, and improving fault detection efficiency
- Summary
- Abstract
- Description
- Claims
- Application Information
AI Technical Summary
Problems solved by technology
Method used
Image
Examples
specific Embodiment approach 1
[0041] Specific implementation mode one: refer to figure 1 with Figure 4 This embodiment is specifically described. The deep learning-based method for detecting the loss of fastening bolts at the end of the cross bar described in this embodiment includes:
[0042] Step 1: Obtain high-definition line array images of the fastening bolts at the end of the cross bar, and establish a sample data set;
[0043] Step 2: Perform data amplification on the sample data set;
[0044] Step 3: Mark the images in the dataset;
[0045] Step 4: Establish a TensorFlow Object Detection API deep learning object detection model, and generate a dataset from the original image and labeled data for model training;
[0046] Step 5: Read the passing-car image on the server of the TFDS truck image detection system, roughly locate the sub-image of the fastening bolt part area, and input the sub-image into the trained model by calling the sub-image for real-time fault detection. The probability that t...
PUM
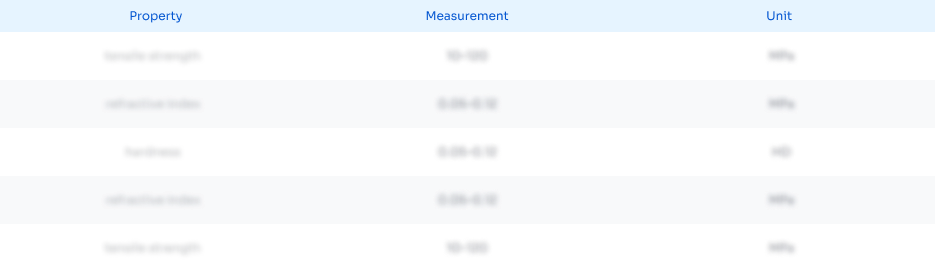
Abstract
Description
Claims
Application Information
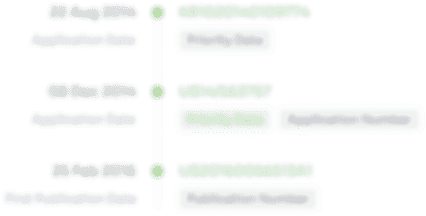
- R&D Engineer
- R&D Manager
- IP Professional
- Industry Leading Data Capabilities
- Powerful AI technology
- Patent DNA Extraction
Browse by: Latest US Patents, China's latest patents, Technical Efficacy Thesaurus, Application Domain, Technology Topic.
© 2024 PatSnap. All rights reserved.Legal|Privacy policy|Modern Slavery Act Transparency Statement|Sitemap