Non-uniform texture small defect detection method based on improved Faster R-CNN model
A detection method and non-uniform technology, which is applied in the field of computer vision, can solve the problems of peak seal foreign matter defects, artificial fatigue, small lithium battery size, etc., achieve good applicability and robustness, meet industrial precision requirements, and discriminate ability Improved effect
- Summary
- Abstract
- Description
- Claims
- Application Information
AI Technical Summary
Problems solved by technology
Method used
Image
Examples
Embodiment Construction
[0051] The technical solutions of the present invention will be clearly and completely described below in conjunction with the accompanying drawings. Apparently, the described embodiments are only some of the embodiments of the present invention, not all of them. Based on the embodiments of the present invention, all other embodiments obtained by persons of ordinary skill in the art without making creative efforts belong to the protection scope of the present invention.
[0052] In the following, the method of the present application will be described in detail by taking the application in the lithium battery to detect the surface defects of the lithium battery as an example.
[0053] The present invention provides a kind of detection method (abbreviation method) of the non-uniform texture small defect based on the improved Faster R-CNN model, specifically comprises the following steps:
[0054] S1. Image acquisition
[0055] Use a high-precision color industrial camera to co...
PUM
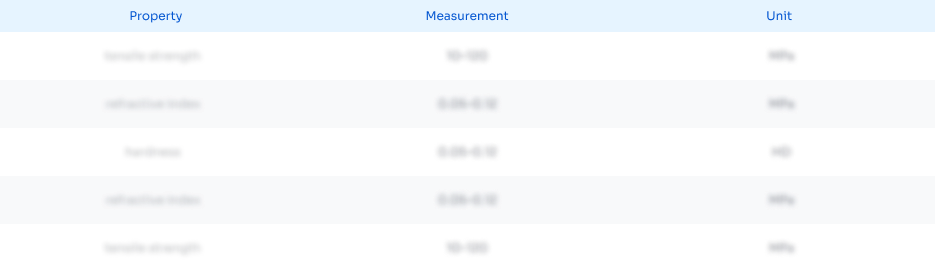
Abstract
Description
Claims
Application Information
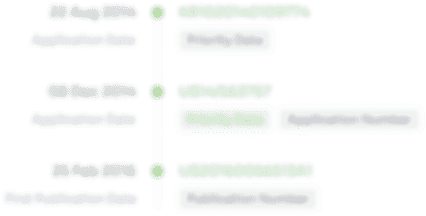
- R&D Engineer
- R&D Manager
- IP Professional
- Industry Leading Data Capabilities
- Powerful AI technology
- Patent DNA Extraction
Browse by: Latest US Patents, China's latest patents, Technical Efficacy Thesaurus, Application Domain, Technology Topic, Popular Technical Reports.
© 2024 PatSnap. All rights reserved.Legal|Privacy policy|Modern Slavery Act Transparency Statement|Sitemap|About US| Contact US: help@patsnap.com