Image super-resolution method based on generative adversarial network
A super-resolution, image-generating technique used in computer vision
- Summary
- Abstract
- Description
- Claims
- Application Information
AI Technical Summary
Problems solved by technology
Method used
Image
Examples
Embodiment
[0089] Image super-resolution methods based on generative confrontation networks, such as figure 2 shown, including the following steps:
[0090] S1. Obtain a training data set and a verification data set;
[0091] In this embodiment, 800 2K images in the DIV2K data set are used to make paired low-resolution-high-resolution images as a training data set; the original 2K images are down-sampled to obtain low-resolution images, which are compared with the original High-resolution images constitute training sample pairs; since the original image size is too large, directly inputting it into the network model for training will cause excessive calculation of the network model and slow down the training speed. Therefore, the training images are randomly cut and the low-resolution The high-resolution image is cropped into an image block of M×K size, where M represents the height of the image block, K represents its width, and the corresponding high-resolution image is cropped into ...
PUM
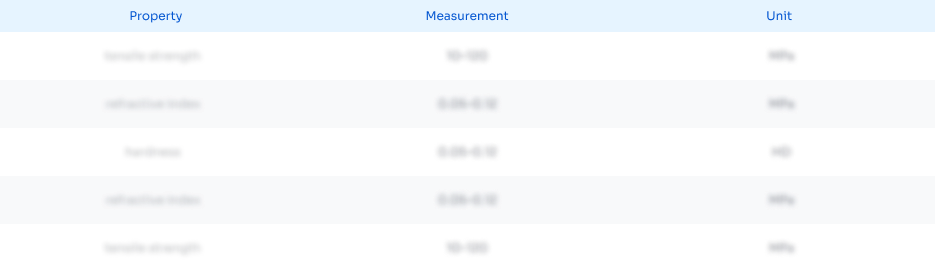
Abstract
Description
Claims
Application Information
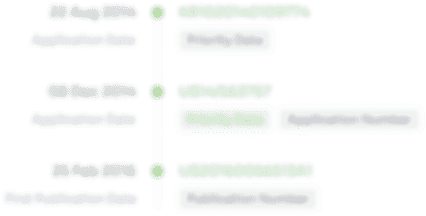
- R&D Engineer
- R&D Manager
- IP Professional
- Industry Leading Data Capabilities
- Powerful AI technology
- Patent DNA Extraction
Browse by: Latest US Patents, China's latest patents, Technical Efficacy Thesaurus, Application Domain, Technology Topic.
© 2024 PatSnap. All rights reserved.Legal|Privacy policy|Modern Slavery Act Transparency Statement|Sitemap