An image super-resolution reconstruction method of a generative adversarial network based on Gaussian coding feedback
A technology of super-resolution reconstruction and feedback network, which is applied in the field of image reconstruction based on Gaussian coding feedback generative confrontation network, which can solve the problems of poor image performance and insufficient edge detail information of the reconstructed image, so as to improve the reconstruction effect and image edge And the effect of clear detail information and better reconstruction effect
- Summary
- Abstract
- Description
- Claims
- Application Information
AI Technical Summary
Problems solved by technology
Method used
Image
Examples
specific Embodiment approach
[0035] Such as figure 1 , 2 As shown in , an image super-resolution reconstruction method based on Gaussian coding feedback to generate adversarial networks, including the following steps:
[0036] A. Preprocess the ImageNet data set to create a reconstruction data set that corresponds one-to-one between low-resolution images and high-resolution images;
[0037] B. Construct a generative confrontation network model for training, and introduce a Gaussian coded feedback network into the model;
[0038] C. Input the data set obtained in step A into the generation confrontation network in turn for model training;
[0039] D. Input the low-resolution image to be processed into the generated network in the trained generation confrontation network to obtain a high-resolution image.
[0040] Further, the steps for making the data set described in step A are:
[0041] A1, obtain the ImageNet data set, randomly select some images as the ImageNet data set;
[0042] A2. Normalize all...
PUM
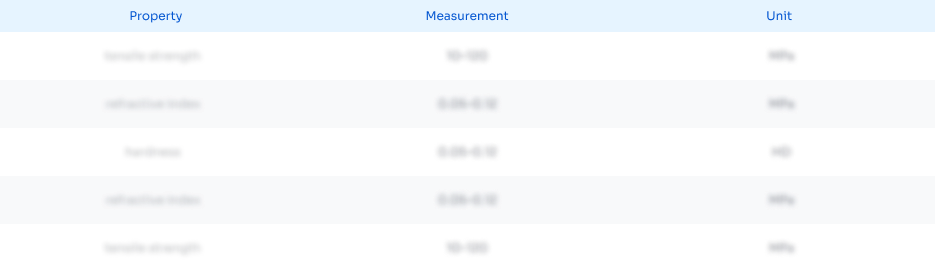
Abstract
Description
Claims
Application Information
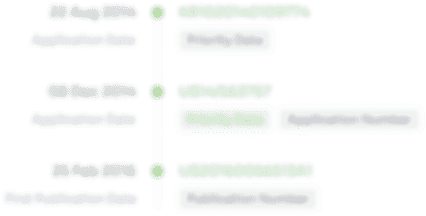
- R&D Engineer
- R&D Manager
- IP Professional
- Industry Leading Data Capabilities
- Powerful AI technology
- Patent DNA Extraction
Browse by: Latest US Patents, China's latest patents, Technical Efficacy Thesaurus, Application Domain, Technology Topic, Popular Technical Reports.
© 2024 PatSnap. All rights reserved.Legal|Privacy policy|Modern Slavery Act Transparency Statement|Sitemap|About US| Contact US: help@patsnap.com