Defect detection semantic segmentation model modeling method and device, medium and equipment
A technology of semantic segmentation and model modeling, applied in neural learning methods, biological neural network models, character and pattern recognition, etc., can solve problems such as low accuracy rate and poor segmentation accuracy, and achieve sufficient features, good model prediction accuracy, The effect of improving the detection rate and segmentation accuracy
- Summary
- Abstract
- Description
- Claims
- Application Information
AI Technical Summary
Problems solved by technology
Method used
Image
Examples
Embodiment 1
[0058] Such as figure 1 As shown, the present application provides a semantic segmentation model modeling method for workpiece defect detection, including the following steps:
[0059] S1 acquires multiple sets of original workpiece point cloud data for training, and the workpiece is a workpiece containing a specified defect type;
[0060] S2 determines the defect composite image of each workpiece based on the original workpiece point cloud data;
[0061] S21 converts the original workpiece point cloud data into a two-dimensional depth map;
[0062] S22 Obtain the first gradient map Scale1 and the second gradient map Scale2 respectively based on the two-dimensional depth map;
[0063] S23 synthesizes a two-dimensional depth map, Scale1, and Scale2 based on the RGB three-channel color image to obtain a defect composite image;
[0064] S3 acquires the defect labeling Label image corresponding to the workpiece:
[0065] S4 Build a semantic segmentation model: set the initiali...
Embodiment 2
[0094] For the defect types of workpieces used for training, one or more types can be preset as required, and the model design can be customized to set the type and number of models, that is, to design a custom defect segmentation network. On the basis of Embodiment 1, this application continues to further design the semantic segmentation model. The deep learning semantic segmentation defect detection model built by this application includes a sequentially connected feature extraction network and feature prediction network;
[0095] The feature extraction network is used to predict the location of the defect area, which includes a feature extraction layer, a feature compression layer, a feature flattening layer, and a feature classification layer;
[0096] Feature extraction is performed on the image data according to the feature extraction layer, and a feature map of the image data is obtained;
[0097] Compress the feature map according to the feature compression layer and o...
Embodiment 3
[0135] On the basis of Embodiment 1 and / or Embodiment 2, corresponding to the above-mentioned training data, the application further limits the input of the semantic segmentation model, and also provides a defect detection method based on the semantic segmentation model. The image of the workpiece to be detected is preprocessed, that is, the input of the semantic segmentation model is the defect composite image of the workpiece to be detected, and after the preprocessing of the defect composite image of the workpiece to be detected, the pb file of the defect prediction model is called to realize the online prediction of the 3D defect of the workpiece And quantification, and the defect form is more intuitive, and better detection results are obtained.
[0136] Such as Figure 4 As shown, the defect detection method based on the semantic segmentation model shown in this embodiment obtains point cloud data of workpiece defects through line laser scanning, then obtains point cloud...
PUM
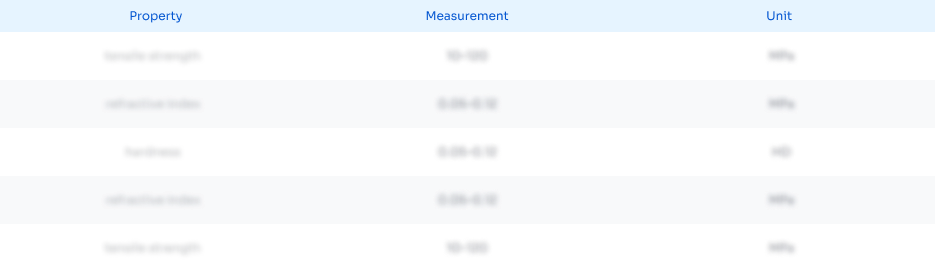
Abstract
Description
Claims
Application Information
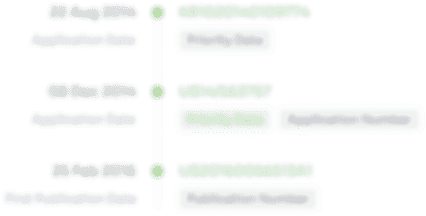
- R&D Engineer
- R&D Manager
- IP Professional
- Industry Leading Data Capabilities
- Powerful AI technology
- Patent DNA Extraction
Browse by: Latest US Patents, China's latest patents, Technical Efficacy Thesaurus, Application Domain, Technology Topic, Popular Technical Reports.
© 2024 PatSnap. All rights reserved.Legal|Privacy policy|Modern Slavery Act Transparency Statement|Sitemap|About US| Contact US: help@patsnap.com