Medical image segmentation method based on lightweight full convolutional neural network
A convolutional neural network and medical image technology, applied in the field of image feature extraction and segmentation, can solve the problem of less research and application of full convolutional neural network model cropping, and achieve the effects of ensuring visual effects, improving computing quality, and ensuring quality
- Summary
- Abstract
- Description
- Claims
- Application Information
AI Technical Summary
Problems solved by technology
Method used
Image
Examples
Embodiment Construction
[0044] The technical solutions in the embodiments of the present invention will be described clearly and in detail below with reference to the drawings in the embodiments of the present invention. The described embodiments are only some of the embodiments of the invention.
[0045] The technical scheme that the present invention solves the problems of the technologies described above is:
[0046] A kind of medical image segmentation method based on lightweight fully convolutional neural network, is characterized in that, comprises the following steps:
[0047] Step 1: Perform preprocessing such as grayscale, normalization, contrast-limited adaptive histogram equalization (CLAHE), and gamma correction (Gamma adjustment) on the data set to improve the segmentation quality of medical images;
[0048] Step 2: Since the amount of preprocessed data in step 1 is too small, in order to effectively avoid overfitting and falling into local optimal solutions during deep network training...
PUM
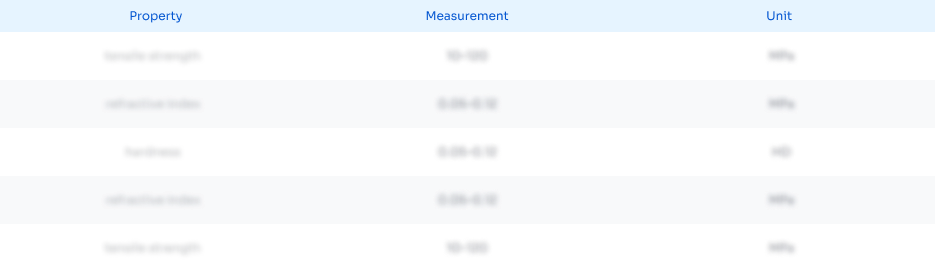
Abstract
Description
Claims
Application Information
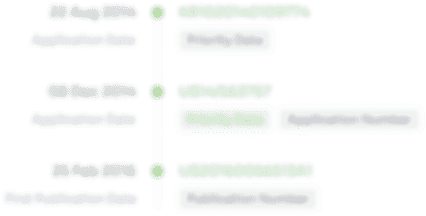
- R&D Engineer
- R&D Manager
- IP Professional
- Industry Leading Data Capabilities
- Powerful AI technology
- Patent DNA Extraction
Browse by: Latest US Patents, China's latest patents, Technical Efficacy Thesaurus, Application Domain, Technology Topic, Popular Technical Reports.
© 2024 PatSnap. All rights reserved.Legal|Privacy policy|Modern Slavery Act Transparency Statement|Sitemap|About US| Contact US: help@patsnap.com