Commodity sequence recommendation method based on deep learning
A recommendation method and deep learning technology, applied in the field of fault handling, can solve problems such as inability to establish long-term dependencies, weight loss, and inability to model two-way commodity sequences
- Summary
- Abstract
- Description
- Claims
- Application Information
AI Technical Summary
Problems solved by technology
Method used
Image
Examples
Embodiment Construction
[0064] The following will clearly and completely describe the technical solutions in the embodiments of the present invention in conjunction with the accompanying drawings in the embodiments of the present invention; obviously, the described embodiments are only part of the embodiments of the present invention, not all embodiments, based on The embodiments of the present invention and all other embodiments obtained by persons of ordinary skill in the art without making creative efforts belong to the protection scope of the present invention.
[0065] see Figure 1 to Figure 3 , a product sequence recommendation method based on deep learning, including the following steps:
[0066] Step 1: Obtain the public product sequence purchased by the user, preprocess it, and obtain the product name and metadata of the product;
[0067] Step 2: The product name and metadata are used as input and sent to the designed sequence recommendation model to obtain a pre-training model. The pre-tr...
PUM
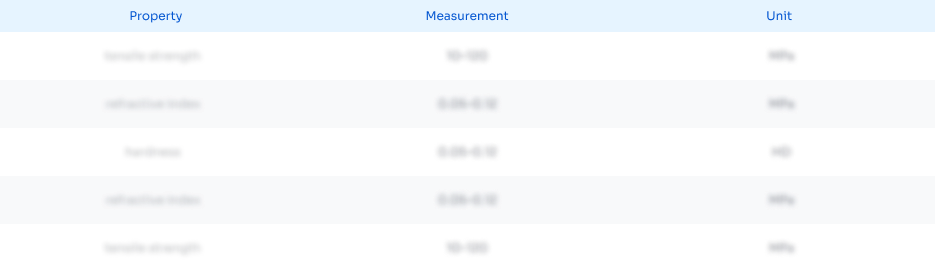
Abstract
Description
Claims
Application Information
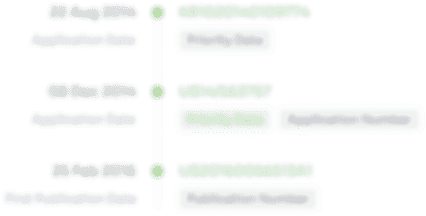
- R&D Engineer
- R&D Manager
- IP Professional
- Industry Leading Data Capabilities
- Powerful AI technology
- Patent DNA Extraction
Browse by: Latest US Patents, China's latest patents, Technical Efficacy Thesaurus, Application Domain, Technology Topic, Popular Technical Reports.
© 2024 PatSnap. All rights reserved.Legal|Privacy policy|Modern Slavery Act Transparency Statement|Sitemap|About US| Contact US: help@patsnap.com