Model training method and device, server and storage medium
A model training and server technology, applied in the field of model training, can solve problems such as expensive, poor generalization performance of algorithm models, difficulties, etc., achieve the effect of alleviating dependence, alleviating model training's dependence on real sampled data, and accelerating application implementation
- Summary
- Abstract
- Description
- Claims
- Application Information
AI Technical Summary
Problems solved by technology
Method used
Image
Examples
Embodiment 1
[0042] figure 1 The flow chart of the model training method provided by Embodiment 1 of the present invention specifically includes the following steps:
[0043] Step 110, sample set IP k Input training model set AP k Perform training to obtain the kth training result, the kth training result includes the training model set AP k+1 and the kth sample label, the kth sample label is a training error label;
[0044] In this embodiment, when the k=1, the sample set IP k is the initial sample, the training model set AP k for the initial training model. In some embodiments, the initial training model and initial samples can also be generated in a completely random manner, or the initial model and initial samples can be heuristically generated using prior knowledge, and the one-time generation does not exceed N AP An arbitrary positive integer number of initial training models and no more than N AP An arbitrary positive integer number of initial samples.
[0045] In this embod...
Embodiment 2
[0057] figure 2 The flow chart of the model training method provided by Embodiment 2 of the present invention specifically includes the following steps:
[0058] Step 210, sample set IP k Input training model set AP k Perform training to obtain the kth training result, the kth training result includes the training model set AP k+1 and the kth sample label, the kth sample label is a training error label;
[0059] In this embodiment, when the k=1, the sample set IP k is the priori known initial sample, the training model set AP k is the priori known initial training model. In some embodiments, the initial training model and initial samples may also be generated in a completely random manner.
[0060] In this embodiment, the sample labeling refers to labeling the samples by using the size of the error during training during the training process of the samples. In this embodiment, the calibrated error is the ratio of misclassified samples of the training model set during t...
Embodiment 3
[0076] The model training device provided by the embodiment of the present invention can execute the model training method provided by any embodiment of the present invention, see image 3 , the model training device 3 includes a training module 31 , a target sample set confirmation module 32 and a sample set generation module 33 .
[0077] Training module 31 is used for sample set IP k Input training model set AP k Perform training to obtain the kth training result, the kth training result includes the training model set AP k+1 and the kth sample label, the kth sample label is a training error label;
[0078] In this embodiment, when the k=1, the sample set IP k is the priori known initial sample, the training model set AP k is the priori known initial training model.
[0079] The target sample set confirmation module 32 is used to confirm the sample set IP according to the kth sample label k Target sample set IP in n ;
[0080] In this embodiment, specifically, the t...
PUM
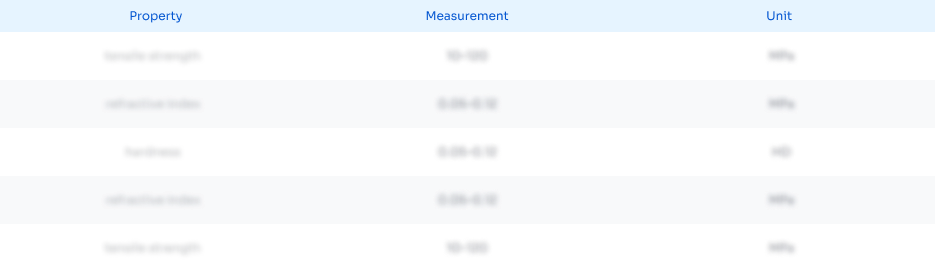
Abstract
Description
Claims
Application Information
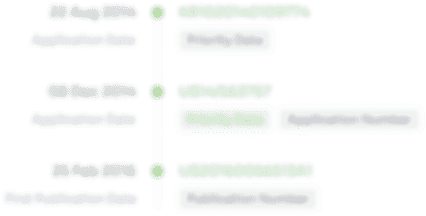
- R&D Engineer
- R&D Manager
- IP Professional
- Industry Leading Data Capabilities
- Powerful AI technology
- Patent DNA Extraction
Browse by: Latest US Patents, China's latest patents, Technical Efficacy Thesaurus, Application Domain, Technology Topic, Popular Technical Reports.
© 2024 PatSnap. All rights reserved.Legal|Privacy policy|Modern Slavery Act Transparency Statement|Sitemap|About US| Contact US: help@patsnap.com