Templated deployment method for machine learning model and custom operator
A machine learning model and a custom technology, applied in the field of deployment of machine learning models and custom operators, can solve the problems of incompatibility with all models, cumbersome, weak support, etc., to reduce personnel development costs and time costs, and shorten deployment the effect of time
- Summary
- Abstract
- Description
- Claims
- Application Information
AI Technical Summary
Problems solved by technology
Method used
Image
Examples
Embodiment Construction
[0035] like figure 1 The templated deployment method of the machine learning model and the self-defined operator of the present invention is shown, including:
[0036]A. According to the prediction process of the machine learning model, the processor of the server sets in the storage medium a general template with 5 steps for calling when different framework models are instantiated, wherein the 5 steps are: input detection, Model loading, data transformation, prediction results and unloading models. In the general template, there are common codes and non-common parts that implement the above five steps. The general codes include: data receiving, data parsing, field inspection, result packaging, etc., and the non-common parts include: environment dependency introduction, model ID, model Reading, data transformation, model prediction, etc. Replace the non-common parts described in the form of substitution symbols.
[0037] Since there may be non-standard code writing or custo...
PUM
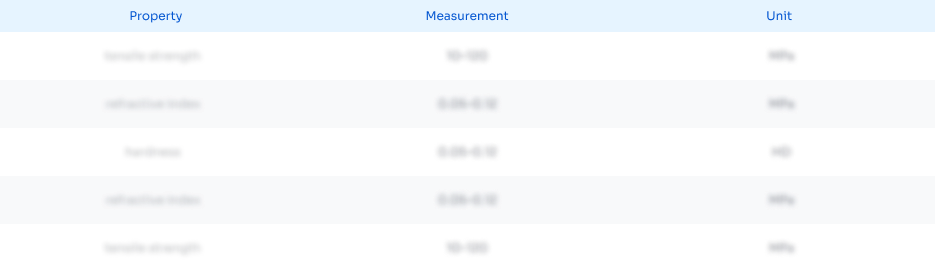
Abstract
Description
Claims
Application Information
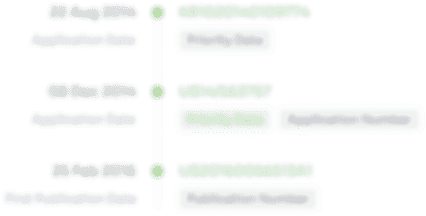
- R&D Engineer
- R&D Manager
- IP Professional
- Industry Leading Data Capabilities
- Powerful AI technology
- Patent DNA Extraction
Browse by: Latest US Patents, China's latest patents, Technical Efficacy Thesaurus, Application Domain, Technology Topic.
© 2024 PatSnap. All rights reserved.Legal|Privacy policy|Modern Slavery Act Transparency Statement|Sitemap