Object reciprocating motion distance evaluation method based on deep learning
A reciprocating motion and deep learning technology, applied in biological neural network models, neural architecture, design optimization/simulation, etc., can solve problems such as calculation errors, distance measurement occlusion, unreliability, etc., to increase credibility, compensate for errors, The result is precise effect
- Summary
- Abstract
- Description
- Claims
- Application Information
AI Technical Summary
Problems solved by technology
Method used
Image
Examples
Embodiment 1
[0044] refer to figure 1 , implement chest compression distance assessment, the main steps are as follows:
[0045] Step S1: Parameter initialization, initializing the window length, filtering parameters and mutation threshold.
[0046] Step S2: Software deviation correction. When there are initialization parameters outside the reasonable range, use software deviation correction to correct the parameters.
[0047] Step S3: Data sampling. The compression displacement detection device is installed according to the requirements of the experiment. The acceleration sensor collects the acceleration changes of the chest compression movement. The data is recorded in the internal memory and the SD card at the same time, and the sampling data can be obtained by reading the SD card.
[0048] Step S4: filter processing, including the following steps:
[0049] S4.1. Use a low-pass amplitude-controlled filter with a filter parameter of 0.05-0.3 to filter inappropriate white noise and hamm...
PUM
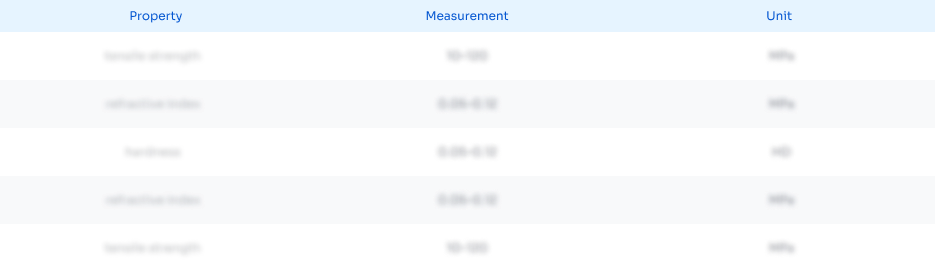
Abstract
Description
Claims
Application Information
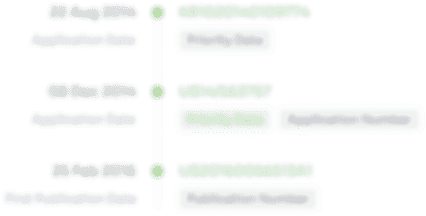
- Generate Ideas
- Intellectual Property
- Life Sciences
- Materials
- Tech Scout
- Unparalleled Data Quality
- Higher Quality Content
- 60% Fewer Hallucinations
Browse by: Latest US Patents, China's latest patents, Technical Efficacy Thesaurus, Application Domain, Technology Topic, Popular Technical Reports.
© 2025 PatSnap. All rights reserved.Legal|Privacy policy|Modern Slavery Act Transparency Statement|Sitemap|About US| Contact US: help@patsnap.com