Deep learning image denoising method integrating multiple scales and attention mechanism
A technology of deep learning and attention, applied in neural learning methods, image enhancement, image analysis, etc., can solve problems such as single network structure, rich image ratio, and multiple mechanisms not used in combination, so as to achieve rapid training and good recovery Image detail, restored image detail rich effect
- Summary
- Abstract
- Description
- Claims
- Application Information
AI Technical Summary
Problems solved by technology
Method used
Image
Examples
Embodiment Construction
[0035] Below in conjunction with accompanying drawing, the scheme of the present invention is described in further detail:
[0036] figure 1 It is the overall flowchart of the scheme of the present invention, which is divided into four steps, making a paired data set of clean images and noise images, building a deep neural network model that integrates multi-scale and attention mechanisms, selecting a suitable optimizer and loss function for training, and finally Input the image to be denoised in the test set into the trained network to obtain the denoised image.
[0037] figure 2 It is a schematic diagram of the overall deep learning network structure. Two Ugroup cascades are used for feature extraction. Ugroup is composed of U-Net and dense residual blocks. The features extracted by the two Ugroups are superimposed and fused through the CBAM attention mechanism module.
[0038] image 3 It is a schematic diagram of the Ugroup structure. Each scale consists of a 3×3 convo...
PUM
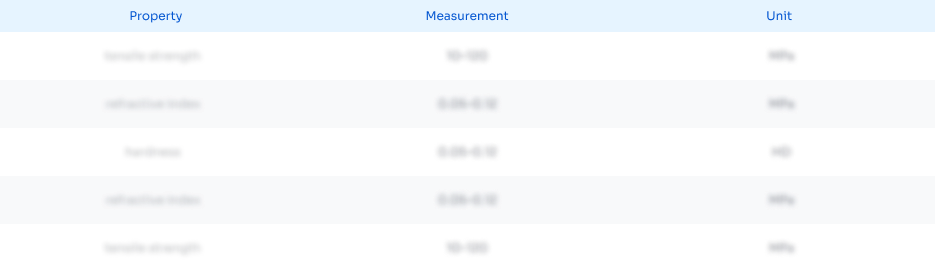
Abstract
Description
Claims
Application Information
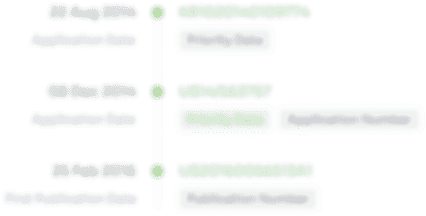
- R&D Engineer
- R&D Manager
- IP Professional
- Industry Leading Data Capabilities
- Powerful AI technology
- Patent DNA Extraction
Browse by: Latest US Patents, China's latest patents, Technical Efficacy Thesaurus, Application Domain, Technology Topic, Popular Technical Reports.
© 2024 PatSnap. All rights reserved.Legal|Privacy policy|Modern Slavery Act Transparency Statement|Sitemap|About US| Contact US: help@patsnap.com