Vessel Segmentation Method of Fundus Image Based on Multi-scale Features of Fully Convolutional Neural Network
A convolutional neural network and multi-scale feature technology, applied in neural learning methods, biological neural network models, image analysis, etc., can solve the problem that neural networks cannot segment blood vessel images well, have multiple additional conditions, and require high image quality, etc. problem, to achieve the effect of avoiding the image processing process
- Summary
- Abstract
- Description
- Claims
- Application Information
AI Technical Summary
Problems solved by technology
Method used
Image
Examples
Embodiment Construction
[0014] The present invention will be further described below in combination with schematic diagrams.
[0015] refer to figure 1 and figure 2 , a blood vessel segmentation method for fundus images based on multi-scale features of fully convolutional neural networks, including the following steps:
[0016] 1) Image preprocessing
[0017] The quality of the image directly affects the accuracy of the effect. The purpose of image preprocessing is mainly to eliminate irrelevant information in the image, simplify the data to the greatest extent, and overcome image interference. First, assign different weights to the R, G, and B channel values of each color retinal image according to the formula Gray=R*0.299+G*0.587+B*0.114, and convert the image into a single-channel grayscale image. Normalization is then performed to improve the contrast and clarity of blood vessels in the picture. Finally, Gamma correction is performed to enhance the contrast of the image. After preprocessi...
PUM
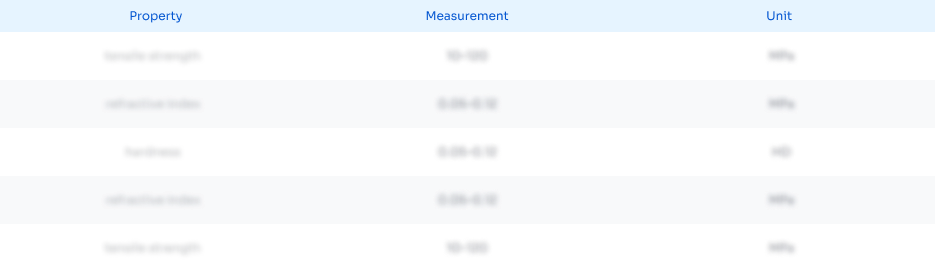
Abstract
Description
Claims
Application Information
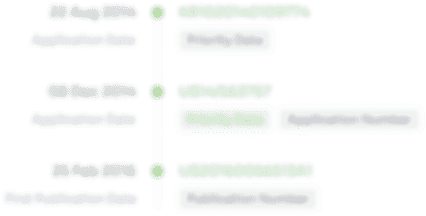
- R&D Engineer
- R&D Manager
- IP Professional
- Industry Leading Data Capabilities
- Powerful AI technology
- Patent DNA Extraction
Browse by: Latest US Patents, China's latest patents, Technical Efficacy Thesaurus, Application Domain, Technology Topic, Popular Technical Reports.
© 2024 PatSnap. All rights reserved.Legal|Privacy policy|Modern Slavery Act Transparency Statement|Sitemap|About US| Contact US: help@patsnap.com