A RGBD Salient Object Detection Method Based on Siamese Network
A twin network and object detection technology, applied in the fields of image processing and computer vision, can solve the problems of difficult training, complex models, and unfavorable mining of the commonality of RGB maps and depth maps, so as to improve the detection performance and reduce the number of training data. Effect
- Summary
- Abstract
- Description
- Claims
- Application Information
AI Technical Summary
Problems solved by technology
Method used
Image
Examples
Embodiment 1
[0042] A RGBD salient object detection method based on twin network, the flow chart is as follows figure 1 As shown, it specifically includes the following steps:
[0043] S1: Prepare training pictures required for training. Wherein, according to the RGBD saliency detection task involved in the present invention, the training pictures include the original RGB image, the corresponding depth image, and the corresponding expected saliency image. The original RGB image and the depth image (Depth) are used as the network input, and the expected saliency map is used as the expectation of the network output, which is used to calculate the loss function and optimize the network.
[0044] S2: Design twin neural network structure and decoder with fusion function, including:
[0045]S2-1: Design the Siamese neural network part. The twin network is actually implemented by two parallel networks with all parameters shared and consistent structure, which can be VGG-16 structure, Resnet-50...
Embodiment 2
[0056] In this embodiment, the twin neural network part is based on the common VGG-16 network structure, and its Conv1_1-Pool5 part is taken, which is divided into a main network and a side channel, including a total of 13 convolutional layers and 6 levels. From top to bottom are Conv1_1~1_2, Conv2_1~2_2, Conv3_1~3_3, Conv4_1~4_3, Conv5_1~5_3, Pool5. The input resolution of the main network is 320×320, and the output resolution is 20×20. In addition, there are 6 side channels (side channel 1-side channel 6), which are respectively connected to the output of the 6 levels of the main network, namely Conv1_2, Conv2_2, Conv3_3, Conv4_3, Conv5_3, Pool5, and each side channel consists of 2 layers of convolution The output resolutions of the side channels from shallow to deep and from top to bottom are 320×320 (side channel 1), 160×160 (side channel 2), 80×80 (side channel 3), 40×40 ( Side channel 4), 20×20 (side channel 5), 20×20 (side channel 6), the network structure diagram is a...
PUM
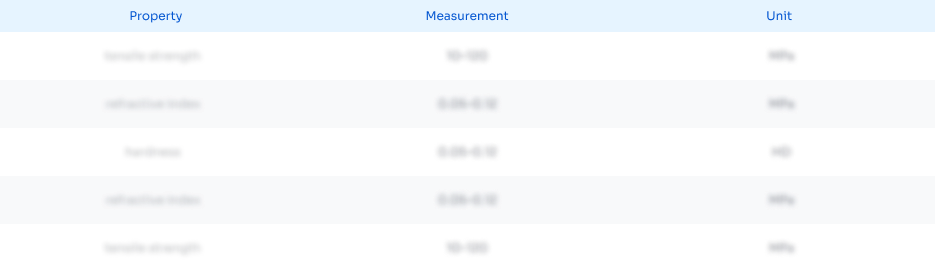
Abstract
Description
Claims
Application Information
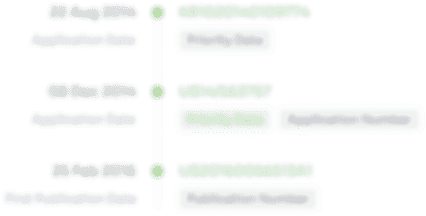
- R&D Engineer
- R&D Manager
- IP Professional
- Industry Leading Data Capabilities
- Powerful AI technology
- Patent DNA Extraction
Browse by: Latest US Patents, China's latest patents, Technical Efficacy Thesaurus, Application Domain, Technology Topic, Popular Technical Reports.
© 2024 PatSnap. All rights reserved.Legal|Privacy policy|Modern Slavery Act Transparency Statement|Sitemap|About US| Contact US: help@patsnap.com