Ship flow prediction method based on deep learning
A technology of ship flow and prediction method, which is applied in the field of ship flow prediction of IF-CEEMD-LSTM, which can solve the problems of low time series prediction accuracy, disappearance of the gradient of the recurrent neural network, and the model cannot meet the prediction accuracy.
- Summary
- Abstract
- Description
- Claims
- Application Information
AI Technical Summary
Problems solved by technology
Method used
Image
Examples
Embodiment Construction
[0068] To make the purpose, technical solutions and advantages of the embodiments of the present invention more clear, the technical solutions in the embodiments of the present invention will be clearly and completely described below in conjunction with the accompanying drawings in the embodiments of the present invention. Obviously, the described embodiments are only It is a part of embodiments of the present invention, but not all embodiments. Based on the embodiments of the present invention, all other embodiments obtained by persons of ordinary skill in the art without making creative efforts belong to the protection scope of the present invention.
[0069] The present invention provides a ship traffic forecasting method based on the improved IF-CEEMD-LSTM (isolated forest-complementary ensemble empirical mode decomposition-long short-term memory neural network), first removes the abnormal components in the original ship data with the forest isolation algorithm Post-normal...
PUM
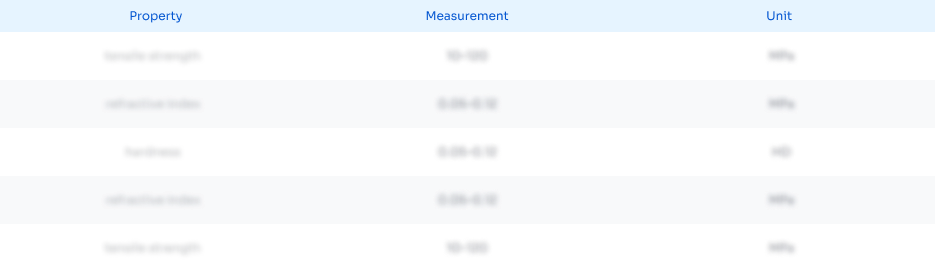
Abstract
Description
Claims
Application Information
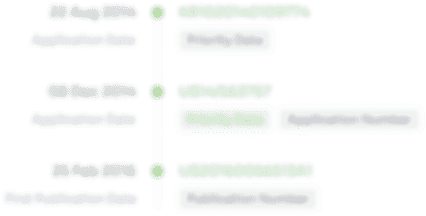
- R&D Engineer
- R&D Manager
- IP Professional
- Industry Leading Data Capabilities
- Powerful AI technology
- Patent DNA Extraction
Browse by: Latest US Patents, China's latest patents, Technical Efficacy Thesaurus, Application Domain, Technology Topic, Popular Technical Reports.
© 2024 PatSnap. All rights reserved.Legal|Privacy policy|Modern Slavery Act Transparency Statement|Sitemap|About US| Contact US: help@patsnap.com