A method for classification and detection of faults in wind turbine components
A wind turbine and fault classification technology, which is applied to computer components, neural learning methods, instruments, etc., can solve problems that affect the accuracy and accuracy of fault prediction, insufficient clarity of data time series, and large amount of data, etc., to improve Accuracy of fault classification, avoidance of deep damage, and high precision
- Summary
- Abstract
- Description
- Claims
- Application Information
AI Technical Summary
Problems solved by technology
Method used
Image
Examples
Embodiment Construction
[0030] The present invention is a fault classification and detection method for wind turbine components. The core of the method is a method for deep mining and feature extraction for wind power SCADA data to classify and detect wind turbine components, in order to improve the accuracy of wind turbine fault detection results. By using the Long Short-Term Memory neural network (Long Short-Term Memory, hereinafter referred to as LSTM neural network) to deeply mine the inherent temporal correlation of the original data, and using the multi-scale characteristics of the wavelet to achieve the extraction of local features of the signal, the decomposition The latter multi-scale signal and the original signal are input to the LSTM neural network for feature extraction, and then the variable adaptive dynamic fusion in the time dimension is carried out, so as to achieve dynamic weighting processing between a variable after global and local feature extraction. The multi-time scale features...
PUM
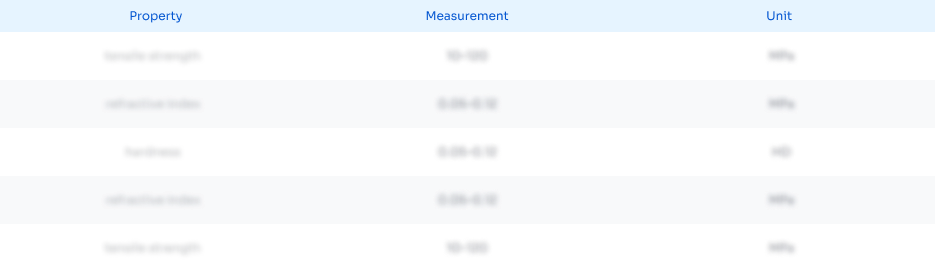
Abstract
Description
Claims
Application Information
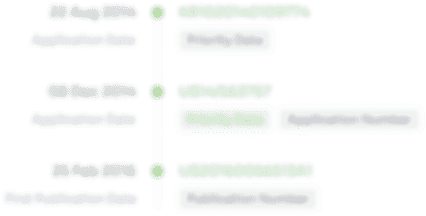
- R&D Engineer
- R&D Manager
- IP Professional
- Industry Leading Data Capabilities
- Powerful AI technology
- Patent DNA Extraction
Browse by: Latest US Patents, China's latest patents, Technical Efficacy Thesaurus, Application Domain, Technology Topic, Popular Technical Reports.
© 2024 PatSnap. All rights reserved.Legal|Privacy policy|Modern Slavery Act Transparency Statement|Sitemap|About US| Contact US: help@patsnap.com