Wireless network resource allocation method based on generative adversarial reinforcement learning
A technology of wireless network resources and allocation method, applied in the field of wireless network resource allocation based on generative confrontation reinforcement learning, to achieve the effect of improving wireless network performance
- Summary
- Abstract
- Description
- Claims
- Application Information
AI Technical Summary
Problems solved by technology
Method used
Image
Examples
Embodiment
[0085] On the host with the configuration shown in Table 1, a simulation environment is written in Python language, and three different types of services (calling, video and ultra-reliable and low-latency services) are used as examples for testing. The resources to be allocated are wireless bandwidth, the total bandwidth is 10M, and the allocation granularity is 1M, so there are 36 allocation strategies in total, that is, the number of valid actions is 36. The discount factor γ is set to 0.9, the number of samples N for sampling the overall return distribution is 50, and the initial value of ∈ is 0.9, which decreases by 0.05 every 100 runs of the algorithm, and remains unchanged when it decreases to 0.05. buffer size N B is 10000. The G network has 3 neurons in the input layer, 512 neurons in the first hidden layer, 512 neurons in the second hidden layer, and 1800 neurons in the output layer. The D network has 50 neurons in the input layer, 256 neurons in the first hidden ...
PUM
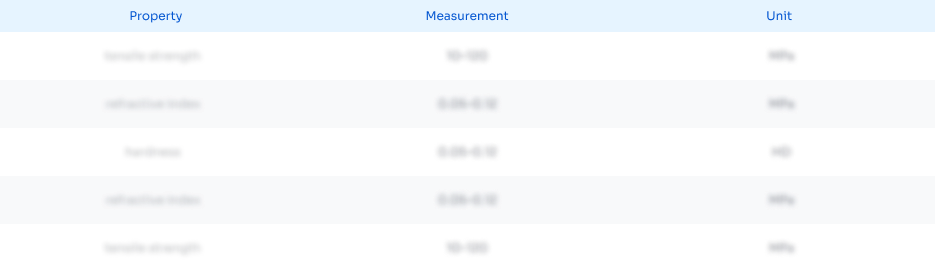
Abstract
Description
Claims
Application Information
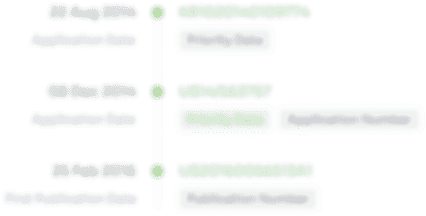
- R&D Engineer
- R&D Manager
- IP Professional
- Industry Leading Data Capabilities
- Powerful AI technology
- Patent DNA Extraction
Browse by: Latest US Patents, China's latest patents, Technical Efficacy Thesaurus, Application Domain, Technology Topic, Popular Technical Reports.
© 2024 PatSnap. All rights reserved.Legal|Privacy policy|Modern Slavery Act Transparency Statement|Sitemap|About US| Contact US: help@patsnap.com