Multi-scale deep convolutional neural network-based satellite image target detection method
A neural network and deep convolution technology, applied in the field of satellite image target detection based on multi-scale deep convolutional neural network, can solve the problems of unsatisfactory detection effect, lack of generalization ability of image distribution, small satellite image objects, etc. Detection results and the ability to distinguish different objects, improved detection results, and strong robustness
- Summary
- Abstract
- Description
- Claims
- Application Information
AI Technical Summary
Problems solved by technology
Method used
Image
Examples
Embodiment Construction
[0042] The specific implementation manner and working principle of the present invention will be further described in detail below in conjunction with the accompanying drawings.
[0043] Such as figure 1 As shown, a satellite image target detection method based on multi-scale deep convolutional neural network, the specific steps are as follows:
[0044] Step 1: Collect satellite image training data sets and perform sample labeling;
[0045] In this example, the samples marked by the sample include a car sample dataset, a building plane sample dataset, an airplane sample dataset, a ship sample dataset and an airport sample dataset, wherein:
[0046] The car sample data set adopts the COWC data set, based on the GSD scale of 15cm, the image is processed by Gaussian check, and a frame of 3m is marked for each car on the GSD scale of 30cm, and a total of 13303 samples are marked;
[0047] The architectural plane sample data set adopts the SpaceNet data set, and the samples are m...
PUM
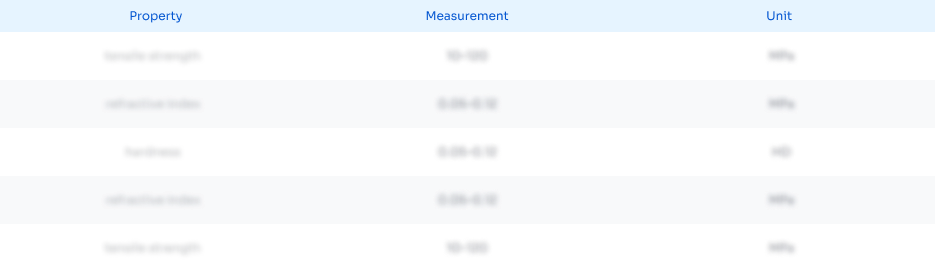
Abstract
Description
Claims
Application Information
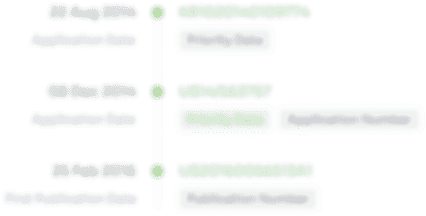
- R&D Engineer
- R&D Manager
- IP Professional
- Industry Leading Data Capabilities
- Powerful AI technology
- Patent DNA Extraction
Browse by: Latest US Patents, China's latest patents, Technical Efficacy Thesaurus, Application Domain, Technology Topic, Popular Technical Reports.
© 2024 PatSnap. All rights reserved.Legal|Privacy policy|Modern Slavery Act Transparency Statement|Sitemap|About US| Contact US: help@patsnap.com