Fundus image segmentation method of full convolutional neural network based on Attention mechanism
A convolutional neural network and fundus image technology, applied in the field of medical image processing, can solve the problems of loss of useful information, inability to fully describe the optic disk of the fundus image, etc., and achieve the effect of improving accuracy and learning ability
- Summary
- Abstract
- Description
- Claims
- Application Information
AI Technical Summary
Problems solved by technology
Method used
Image
Examples
Embodiment Construction
[0046] The present invention is further described in conjunction with the following examples.
[0047] see figure 1 According to the first aspect, the embodiment of the present invention provides a fundus image segmentation method based on a fully convolutional neural network based on an Attention mechanism.
[0048] The method includes the following steps:
[0049] S1 selects the fundus retinal image data as the training set and the test set, and each fundus retinal image sample includes the original color fundus retinal image and its corresponding cup-and-disk segmentation label. Specifically, the cup-and-disc segmentation data of retinal images obtained from clinics are used as the training set to train the model, and the public 400 test data are used as the test set to test and evaluate the performance of the model.
[0050] S2 preprocesses the fundus retinal images in the training set, and uses them to input the fully convolutional neural network model. In an achievabl...
PUM
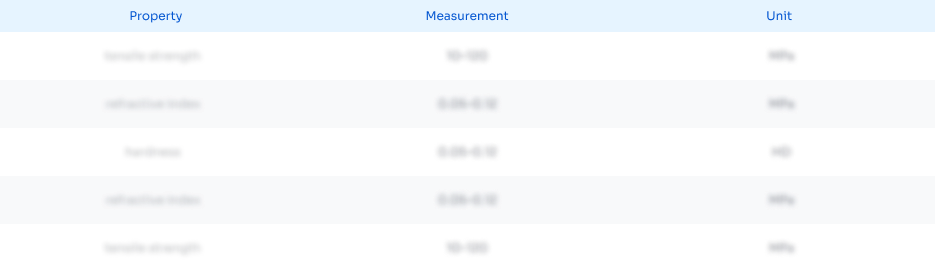
Abstract
Description
Claims
Application Information
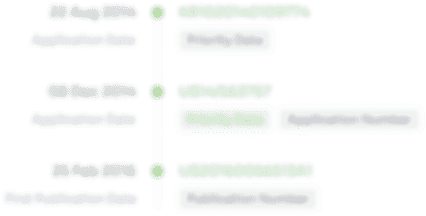
- R&D Engineer
- R&D Manager
- IP Professional
- Industry Leading Data Capabilities
- Powerful AI technology
- Patent DNA Extraction
Browse by: Latest US Patents, China's latest patents, Technical Efficacy Thesaurus, Application Domain, Technology Topic, Popular Technical Reports.
© 2024 PatSnap. All rights reserved.Legal|Privacy policy|Modern Slavery Act Transparency Statement|Sitemap|About US| Contact US: help@patsnap.com