Smoke detection method of integrated convolutional neural network
A convolutional neural network and detection method technology, applied in the field of smoke identification, can solve the problems of high smoke monitoring cost, insufficient accuracy and low efficiency, and achieve the effects of reducing smoke detection cost, improving accuracy and saving capital.
- Summary
- Abstract
- Description
- Claims
- Application Information
AI Technical Summary
Problems solved by technology
Method used
Image
Examples
Embodiment 1
[0044] Such as figure 1 , 4 As shown in ~5, a smoke detection method integrating convolutional neural network, including a suspected smoke acquisition module, a suspected smoke confirmation module and a smoke alarm module, the specific implementation steps are:
[0045] S1. The suspected smoke acquisition module acquires images containing suspected smoke after the real-time acquired images are detected by the Faster R-CNN model;
[0046] S2. The suspected smoke detection module uses the convolutional neural network to detect the candidate area of the suspected smoke image, determines the image containing smoke, and uses the convolutional neural network (CNN) model to determine the size and position of the candidate area according to the center of the candidate area as a new candidate The center of the candidate area is regenerated, and then the new candidate area is identified by the convolutional neural network. Figure 4 Smoke recognition was performed on the Faster R-CN...
Embodiment 2
[0049]On the basis of the original Faster R-CNN model, the network structure is simplified, and the original 13-layer convolution and 13-layer ReLU functions are reduced to 10-layer convolution and 10-layer ReLU functions. After the reduction, the effect of feature extraction remains unchanged. , but the amount of calculation becomes smaller, which reduces the overall false positives and improves the accuracy.
[0050] After being processed by Faster R-CNN, the suspected smoke area is obtained, and the area selected by the frame on the image is the suspected smoke area. Then, the convolutional neural network is used to determine whether there is smoke in the suspected smoke image, and finally the result is processed.
Embodiment 3
[0052] Such as figure 2 As shown, the specific implementation steps of the suspected smoke acquisition module in step S1 to obtain images containing suspected smoke after passing the Faster RCNN model detection are as follows:
[0053] S11. Obtain the smoke detection Faster R-CNN model after training through the Faster R-CNN network according to a large number of smoke data samples;
[0054] S12. Use the trained smoke detection Faster R-CNN model to detect real-time video target images, and obtain images of suspected smoke candidate areas;
[0055] S13. According to the step S12, obtain the image containing the suspected smoke candidate frame, and according to the smoke similarity score corresponding to each image, when the score is greater than a certain threshold, it is determined to be smoke.
[0056] The Faster R-CNN network structure is composed of three neural networks including CNN (Convolutional Neural Networks, convolutional neural network), RPN (Region Proposal Net...
PUM
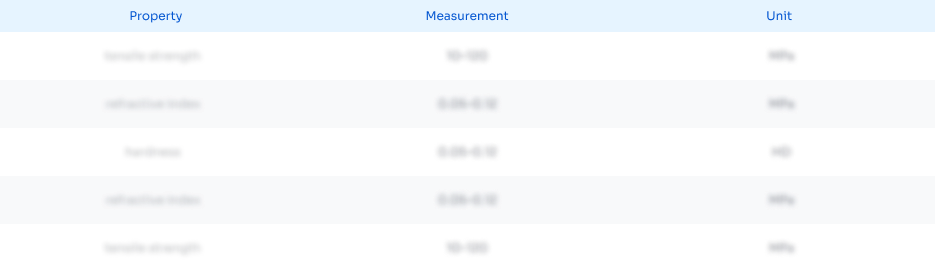
Abstract
Description
Claims
Application Information
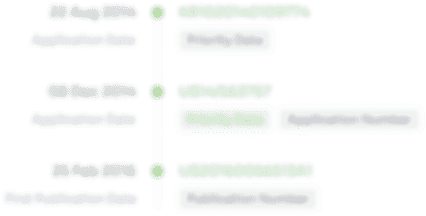
- R&D Engineer
- R&D Manager
- IP Professional
- Industry Leading Data Capabilities
- Powerful AI technology
- Patent DNA Extraction
Browse by: Latest US Patents, China's latest patents, Technical Efficacy Thesaurus, Application Domain, Technology Topic, Popular Technical Reports.
© 2024 PatSnap. All rights reserved.Legal|Privacy policy|Modern Slavery Act Transparency Statement|Sitemap|About US| Contact US: help@patsnap.com