A Width Transfer Learning Network and Rolling Bearing Fault Diagnosis Method Based on Width Transfer Learning Network
A technology of transfer learning and rolling bearings, which is applied in the field of bearing fault diagnosis, can solve problems such as large differences in the distribution of source domain data and target domain data, low diagnostic accuracy and model training efficiency, and unbalanced distribution of multi-state data, so as to reduce training costs. time, shorten training time, and improve accuracy
- Summary
- Abstract
- Description
- Claims
- Application Information
AI Technical Summary
Problems solved by technology
Method used
Image
Examples
Embodiment Construction
[0059] combined with Figures 1 to 10 A width transfer learning network and a rolling bearing fault diagnosis method based on the width transfer learning network of the present invention are described in detail as follows:
[0060] 1 Basic theory
[0061] 1.1 Breadth Learning System (BLS)
[0062] Breadth Learning System (BLS) perfectly inherits random vector functional-link neural network (random vector functional-link neural network, RVFLNN) [26] The advantages of extremely strong nonlinear mapping capabilities, and can process data quickly and efficiently, saving time and improving efficiency. Many neural networks are plagued by time-consuming training, the main reason is that there are a large number of parameters between their layers, resulting in long training cycles and low efficiency, and when the established model does not achieve the desired purpose, it will consume a lot of time again. Retrain. The design of wide learning network provides an effective solution t...
PUM
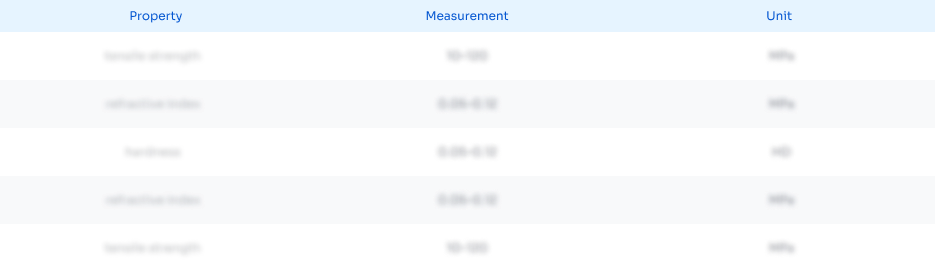
Abstract
Description
Claims
Application Information
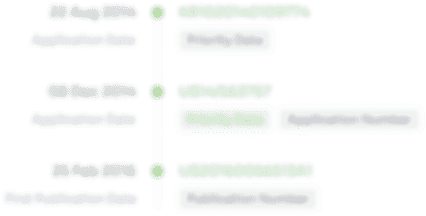
- R&D Engineer
- R&D Manager
- IP Professional
- Industry Leading Data Capabilities
- Powerful AI technology
- Patent DNA Extraction
Browse by: Latest US Patents, China's latest patents, Technical Efficacy Thesaurus, Application Domain, Technology Topic, Popular Technical Reports.
© 2024 PatSnap. All rights reserved.Legal|Privacy policy|Modern Slavery Act Transparency Statement|Sitemap|About US| Contact US: help@patsnap.com