Semi-supervised semantic segmentation method based on maximum confidence
A technology of semantic segmentation and confidence, which is applied in the direction of instruments, biological neural network models, calculations, etc., can solve the problems of weak supervision methods, lack of boundary position information, and ignore misclassified information, so as to improve accuracy and reliable prediction results Effect
- Summary
- Abstract
- Description
- Claims
- Application Information
AI Technical Summary
Problems solved by technology
Method used
Image
Examples
Embodiment Construction
[0021] The technical solutions in the embodiments of the present invention will be clearly and completely described below in conjunction with the accompanying drawings in the embodiments of the present invention. Obviously, the described embodiments are only some of the embodiments of the present invention, not all of them. Based on the embodiments of the present invention, all other embodiments obtained by persons of ordinary skill in the art without making creative efforts belong to the protection scope of the present invention.
[0022] The embodiment of the present invention provides a semi-supervised semantic segmentation method based on maximizing confidence. This method proposes a semi-supervised learning framework, which combines supervised learning and unsupervised learning. The method starts from the confidence of the enhanced class probability map point of view to solve the problem. Meanwhile, more attention is paid to misclassified regions, especially in border reg...
PUM
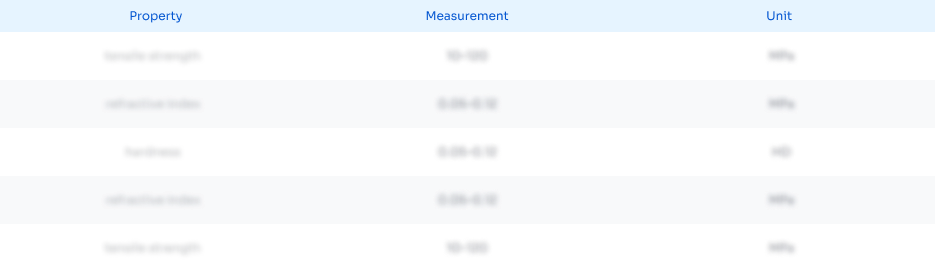
Abstract
Description
Claims
Application Information
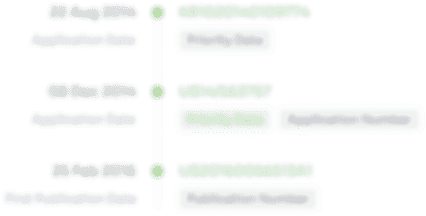
- R&D Engineer
- R&D Manager
- IP Professional
- Industry Leading Data Capabilities
- Powerful AI technology
- Patent DNA Extraction
Browse by: Latest US Patents, China's latest patents, Technical Efficacy Thesaurus, Application Domain, Technology Topic, Popular Technical Reports.
© 2024 PatSnap. All rights reserved.Legal|Privacy policy|Modern Slavery Act Transparency Statement|Sitemap|About US| Contact US: help@patsnap.com