Channel estimation method, device and readable storage medium based on deep neural network
A deep neural network and channel estimation technology, applied to devices and readable storage media, in the field of channel estimation methods based on deep neural networks, can solve problems such as low estimation accuracy, and achieve the effect of improving estimation accuracy
- Summary
- Abstract
- Description
- Claims
- Application Information
AI Technical Summary
Problems solved by technology
Method used
Image
Examples
Embodiment
[0104] The present invention will be described in further detail below in conjunction with the accompanying drawings and embodiments.
[0105]Consider a single-bit quantized single-cell massive MIMO scenario. In this scenario, the channel estimation method based on the deep neural network of the present invention is used to realize uplink channel estimation. The detailed simulation parameters are shown in Table 1.
[0106] Table 1 Simulation parameter table
[0107]
[0108] Comparison scheme
comparative approach 1
[0109] Comparison scheme 1: use the least square method (LS) to estimate the channel. In this scheme, the signal received by the base station is directly estimated by LS without any preprocessing.
comparative approach 2
[0110] Comparison scheme 2: use the linear minimum mean square error estimation method (LMMSE) to estimate the channel. In this scheme, the signal received by the base station is first preprocessed, that is, the Bussgang decomposition method is used to convert the nonlinear quantization process into a linear process, and then Then LMMSE is used to realize channel estimation.
[0111] see Figure 6 and 7 , and plotted the simulation graphs of the estimated performance of four different schemes as the signal-to-noise ratio varies. In this section, two channel models are simulated: one is the Rayleigh block fading channel model; the other is the spatially correlated channel model given by formula (1), it can be seen that the proposed method has the same better than other estimation methods. exist Figure 6 Under the Rayleigh block fading channel model, compared with other algorithms, the proposed algorithm obtains a significant gain of at least 8dB when the SNR is -8dB. also...
PUM
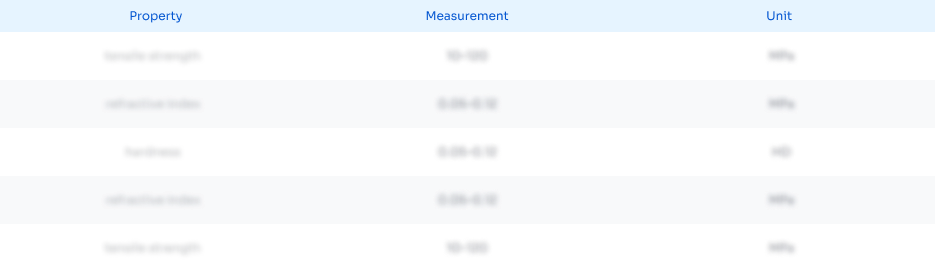
Abstract
Description
Claims
Application Information
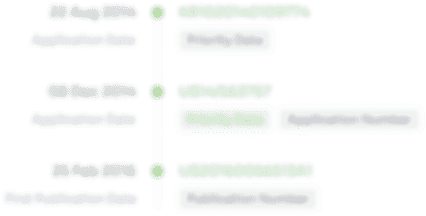
- R&D Engineer
- R&D Manager
- IP Professional
- Industry Leading Data Capabilities
- Powerful AI technology
- Patent DNA Extraction
Browse by: Latest US Patents, China's latest patents, Technical Efficacy Thesaurus, Application Domain, Technology Topic, Popular Technical Reports.
© 2024 PatSnap. All rights reserved.Legal|Privacy policy|Modern Slavery Act Transparency Statement|Sitemap|About US| Contact US: help@patsnap.com