Deep learning-based mobility prediction method of nodes in vehicle-mounted Ad Hoc network
A vehicle-mounted self-organization and deep learning technology, applied in the field of communication, to achieve the effect of accurate prediction
- Summary
- Abstract
- Description
- Claims
- Application Information
AI Technical Summary
Problems solved by technology
Method used
Image
Examples
Embodiment 1
[0025] The flow chart of realizing the node mobility prediction in the vehicle ad hoc network based on deep learning proposed by the present invention is shown in the attached figure 1 Shown, the present invention comprises the following steps:
[0026] Create mobility models for different types of vehicles; combine different types of vehicle movement models to establish a sample database of historical vehicle journey data and a database of traffic law constraints, and agree on the characteristics of the sample journey data; then use the cyclic neural network to extract the deep-level characteristics of the mobility of the vehicle samples, Establish a mobility prediction model; then use the gradient descent backpropagation algorithm to train the model parameters; finally use the real-time data information of the vehicle's current movement to predict the mobility.
Embodiment 2
[0028] A recurrent neural network is used to extract the deep-level characteristics of the mobility of vehicle samples, and a mobility prediction model is established. The problem of gradient disappearance or gradient explosion is prone to occur in the process of solving the simple RNN structure, so that the long-term sequence dependence problem cannot be solved. The present invention mainly adopts a special RNN structure long short-term memory network (Long Short Term Memory, LSTM) that can deal with long-term dependence problems. The structure diagram of the repeating module in its LSTM chain structure is as follows figure 2 shown. Each module corresponds to input x at different times and output h at different times. When training the network, x is the extracted road network information, traffic law constraint information, vehicle driving habit information, vehicle owner information, historical urban road condition information, average traffic speed, vehicle density infor...
PUM
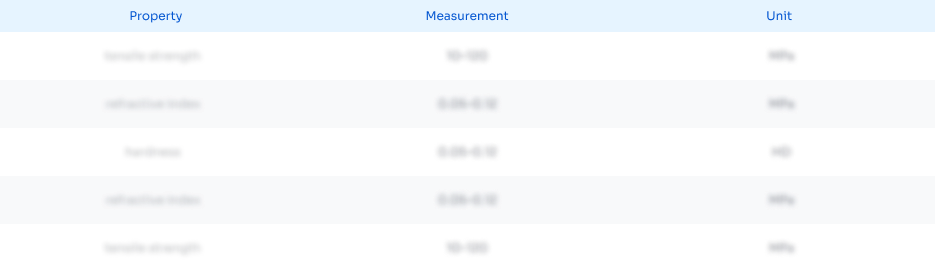
Abstract
Description
Claims
Application Information
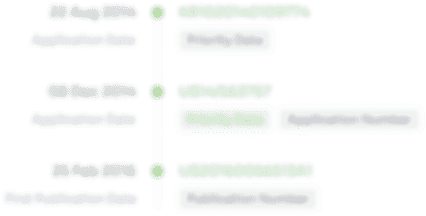
- R&D Engineer
- R&D Manager
- IP Professional
- Industry Leading Data Capabilities
- Powerful AI technology
- Patent DNA Extraction
Browse by: Latest US Patents, China's latest patents, Technical Efficacy Thesaurus, Application Domain, Technology Topic, Popular Technical Reports.
© 2024 PatSnap. All rights reserved.Legal|Privacy policy|Modern Slavery Act Transparency Statement|Sitemap|About US| Contact US: help@patsnap.com