SCSS (Single Channel Speech Separation) algorithm based on DNN (Deep Neural Network)
A deep neural network and speech separation technology, applied in speech analysis, speech recognition, instruments, etc., can solve the problems of speech separation performance impact, time-consuming, poor separation effect, etc., to achieve improved intelligibility, high separation efficiency, and reduced Effect of Distortion Rate
- Summary
- Abstract
- Description
- Claims
- Application Information
AI Technical Summary
Problems solved by technology
Method used
Image
Examples
Embodiment Construction
[0045] In order to make the object, technical solution and advantages of the present invention clearer, the present invention will be described in detail below in conjunction with the accompanying drawings and specific embodiments.
[0046] Such as figure 1 As shown, the present invention provides a kind of single channel speech separation algorithm based on deep neural network, mainly comprises the following steps:
[0047] Step 1: Preprocess the training speech samples and extract their feature information;
[0048] Step 2: Use the loss function to train the deep neural network to obtain the deep neural network model;
[0049] Step 3: Preprocess the speech sample to be tested, extract its feature information, and conduct speech separation through the trained deep neural network model, and then obtain the separation result through speech reconstruction.
[0050] Step 1-Step 3 will be described in detail below.
[0051] Among them, step 1 specifically includes:
[0052] St...
PUM
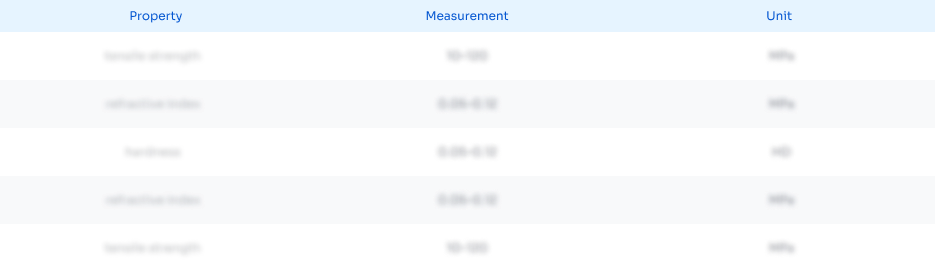
Abstract
Description
Claims
Application Information
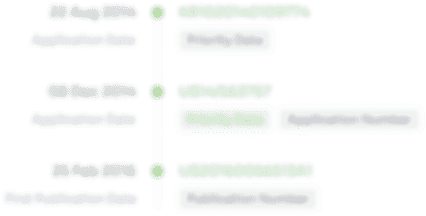
- R&D Engineer
- R&D Manager
- IP Professional
- Industry Leading Data Capabilities
- Powerful AI technology
- Patent DNA Extraction
Browse by: Latest US Patents, China's latest patents, Technical Efficacy Thesaurus, Application Domain, Technology Topic, Popular Technical Reports.
© 2024 PatSnap. All rights reserved.Legal|Privacy policy|Modern Slavery Act Transparency Statement|Sitemap|About US| Contact US: help@patsnap.com