Indoor fusion positioning method based on extended Kalman filtering and particle filtering
An extended Kalman and particle filter technology, applied in the field of wireless sensor networks
- Summary
- Abstract
- Description
- Claims
- Application Information
AI Technical Summary
Problems solved by technology
Method used
Image
Examples
Embodiment 1
[0116] The present invention collects and analyzes data in a laboratory with an area of more than 100 square meters, and the area includes the coverage of WiFi wireless signals. The Huawei P8 (Android 4.0 platform) smartphone was used for data collection.
[0117] Using the signal strength information of WiFi to perform fingerprint positioning, the specific steps are as follows:
[0118] a) collect the wifi signal strength and the coordinates of the known location, and construct a fingerprint database;
[0119] b) use the k-nearest neighbor method to perform fingerprint positioning;
Embodiment 2
[0121] The user walks in a clockwise direction, and collects acceleration, magnetic field strength, and angular velocity with a smartphone.
[0122] By estimating the pedestrian's step length and motion direction, the next step position is calculated. The specific formula is as follows:
[0123]
[0124] The specific steps of pedestrian dead reckoning are as follows:
[0125] a) Using the acceleration differential finite state machine to realize step counting detection;
[0126] b) Estimate the step size with a method based on Kalman filtering;
[0127] c) Fusion direction sensor, gyroscope data to get heading. The formula used for weighted fusion is as follows:
[0128] Ori fuse,t = p · Ori o,t +(1-p) Ori g,t
[0129] In the experiment, the non-linear step size estimation models before and after improvement are used to estimate the step size respectively. Among them, the parameter K of the nonlinear model is taken as 0.35. Obtain the heading angle obtained by the ...
Embodiment 3
[0132] Use the extended Kalman filter algorithm to fuse the WiFi positioning results with the pedestrian dead reckoning positioning results. The specific steps are as follows:
[0133] a) Obtain the prior information of the state according to the system model:
[0134]
[0135] b) using the positioning result of the WiFi fingerprinting method as the observed value of the state vector;
[0136] c) Combine system model and measurement model for status update:
[0137] x k =X k|k-1 +K k (Z k -Z k|k-1 )
[0138] Among them, the initial value of the estimated error covariance of the extended Kalman filter is set to The covariance of the process noise is set to The covariance of the measurement noise is set to
PUM
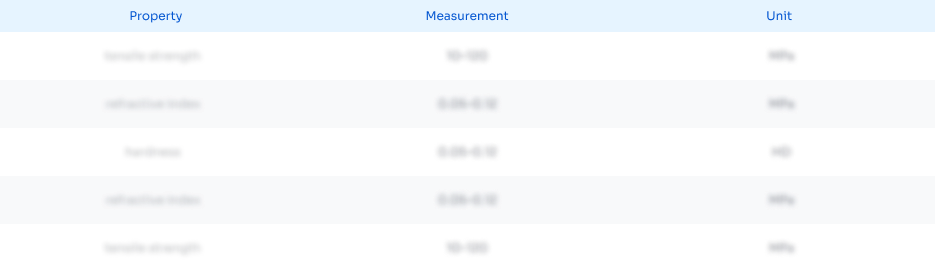
Abstract
Description
Claims
Application Information
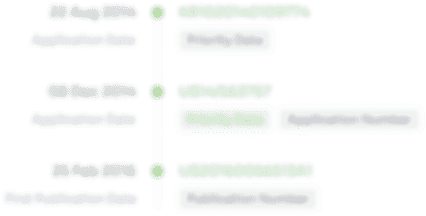
- R&D Engineer
- R&D Manager
- IP Professional
- Industry Leading Data Capabilities
- Powerful AI technology
- Patent DNA Extraction
Browse by: Latest US Patents, China's latest patents, Technical Efficacy Thesaurus, Application Domain, Technology Topic, Popular Technical Reports.
© 2024 PatSnap. All rights reserved.Legal|Privacy policy|Modern Slavery Act Transparency Statement|Sitemap|About US| Contact US: help@patsnap.com