Continuous reinforcement learning system and method based on stochastic differential equation
A stochastic differential equation and reinforcement learning technology, applied in the field of reinforcement learning for continuous systems, can solve problems such as failure to satisfy continuity conditions and uncontrollable variance
- Summary
- Abstract
- Description
- Claims
- Application Information
AI Technical Summary
Problems solved by technology
Method used
Image
Examples
Embodiment Construction
[0045] In order to make the purpose, technical solution and advantages of the present invention clearer, a continuous reinforcement learning system and method based on stochastic differential equations of the present invention will be further described below in conjunction with the accompanying drawings and embodiments.
[0046] The invention proposes a continuous reinforcement learning system and method based on stochastic differential equations, which are suitable for continuous control applications.
[0047] like figure 1 As shown, the step process of a continuous reinforcement learning method based on stochastic differential equations proposed by the present invention includes the following steps:
[0048] Step 1, initialize all parameters in the action policy generator APG, environment state estimator ESE, value estimator VE, memory storage module MS and external environment EE included in the whole learning method.
[0049] The present invention takes the Pendulum-v0 in...
PUM
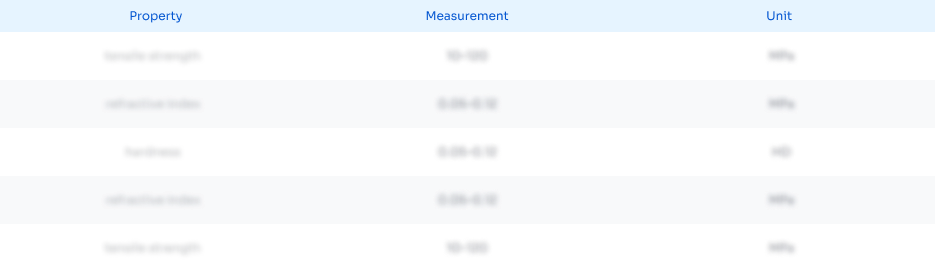
Abstract
Description
Claims
Application Information
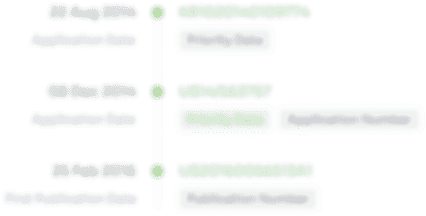
- R&D Engineer
- R&D Manager
- IP Professional
- Industry Leading Data Capabilities
- Powerful AI technology
- Patent DNA Extraction
Browse by: Latest US Patents, China's latest patents, Technical Efficacy Thesaurus, Application Domain, Technology Topic, Popular Technical Reports.
© 2024 PatSnap. All rights reserved.Legal|Privacy policy|Modern Slavery Act Transparency Statement|Sitemap|About US| Contact US: help@patsnap.com