Pseudo CT synthesis method and application based on deep convolutional neural network
A deep convolution and neural network technology, applied in the fields of applications, radiological diagnostic instruments, medical science, etc., can solve problems affecting the prediction accuracy of pseudo-CT, achieve rapid generation and save time
- Summary
- Abstract
- Description
- Claims
- Application Information
AI Technical Summary
Problems solved by technology
Method used
Image
Examples
preparation example Construction
[0047] see Figure 1~4 , the application provides a pseudo-CT synthesis method based on a deep convolutional neural network, the method comprising the steps of:
[0048] Step 1: Collect patient MRI images, CT images and radiotherapy planning information;
[0049] Step 2: the MRI image voxel size is adjusted to be consistent with the CT image voxel size, and then the MRI image and the CT image are carried out image registration;
[0050] Step 3: adopt deep convolutional neural network to set up the correlation model between the MRI image of described registration and CT image, and adopt the mapping relation between MRI voxel intensity value and CT gray value of learning deep convolutional neural network;
[0051] Step 4: Predict pseudo-CT images of patients using the association model.
[0052]Further, in the step 1, the MRI image and the CT image include the MRI image and the CT image of the same part, and the MRI image and the CT image are acquired within a specified time i...
Embodiment 1
[0087] In this example, the pseudo-CT of nasopharyngeal carcinoma patients is generated, and the MRI images and CT images of 45 nasopharyngeal carcinoma patients are selected for model training and testing.
[0088] First, use MATLAB software to process the MRI image, and adjust the voxel size of the MRI image to be consistent with the CT image voxel size. Then use the 3D slicer software to perform rigid registration of the MRI images and CT images of all patients, export the registered MRI images, import them into MATLAB, obtain the masks of all CT images, and use the masks to align all MRI images with CT images. The image background is uniformly set to 0. All MRI voxels and CT voxels intensity distributions are normalized to the range [-1,1]. Select 30 patients as the training set, and perform data enhancement on the MRI images and CT images in the training set. The data enhancement method selected in this example is horizontal / vertical flip and 180° rotation, and export th...
Embodiment 2
[0103] This embodiment is based on the pseudo-CT synthesis method based on the deep convolutional neural network described in the above-mentioned embodiments, and provides a specific application method of the pseudo-CT synthesis method. The specific steps are:
[0104]The pseudo CT generated by the 15 test sets in Example 1 was imported into the Eclipse planning system for dose recalculation, and the setting of planning execution parameters such as the incident field was kept consistent with the clinical actual plan, and the dose distribution of the pseudo CT was compared with the original planned dose distribution. The comparison mode of the dose distribution that the present embodiment takes is mean error (ME), mean absolute error (MAE), gamma evaluation passing rate (2mm / 2%, 3mm / 3%), as shown in table 2:
[0105]
[0106] Table 1 Comparison of the dose distribution based on pseudo-CT recalculation and the real planned dose distribution in the test set
[0107] The result...
PUM
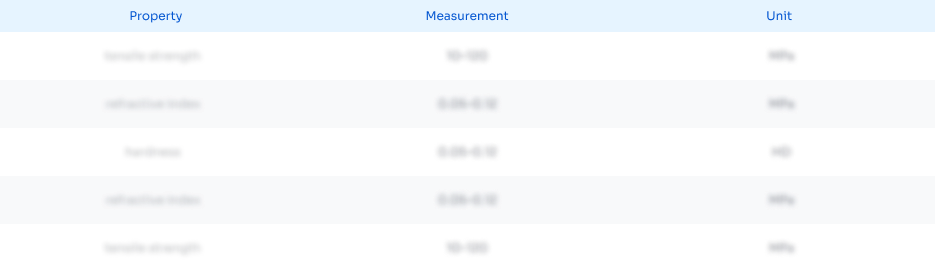
Abstract
Description
Claims
Application Information
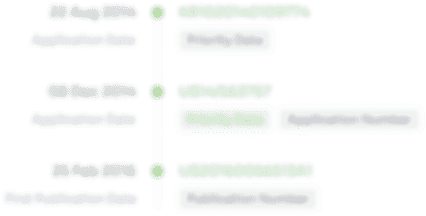
- R&D Engineer
- R&D Manager
- IP Professional
- Industry Leading Data Capabilities
- Powerful AI technology
- Patent DNA Extraction
Browse by: Latest US Patents, China's latest patents, Technical Efficacy Thesaurus, Application Domain, Technology Topic, Popular Technical Reports.
© 2024 PatSnap. All rights reserved.Legal|Privacy policy|Modern Slavery Act Transparency Statement|Sitemap|About US| Contact US: help@patsnap.com