MH-LSTM anomaly detection method based on session feature similarity
An anomaly detection and similarity technology, applied in electrical components, transmission systems, etc., can solve problems such as weak model accuracy, difficulty in depicting data distribution outlines, and decreased detection capabilities, achieving high detection and recall rates
- Summary
- Abstract
- Description
- Claims
- Application Information
AI Technical Summary
Problems solved by technology
Method used
Image
Examples
Embodiment Construction
[0033] The MH-LSTM anomaly detection method of the present invention mainly involves two related technologies. (1) Use the Min-Hash algorithm to extract the features in the session sequence data. (2) Construct LSTM network for abnormal data detection and location.
[0034] (1) Feature extraction in Web session sequence data: Min-Hash algorithm is a simple implementation of the concept of Min-wise Independent Permutation proposed by Broder, and it is a locality-sensitive hashing (Locality-Sensitive Hashing, LSH) kind. The original role of LSH is to efficiently deal with the nearest neighbor problem of massive data and high-dimensional data. LSH maps two data with high similarity to the same hash value with a high probability through a special hash function, and maps two data with low similarity to the same hash value with a very low probability.
[0035] The specific description of Min-Hash is, given a random hash function h(x), the Min-Hash function is defined as m h (υ)=a...
PUM
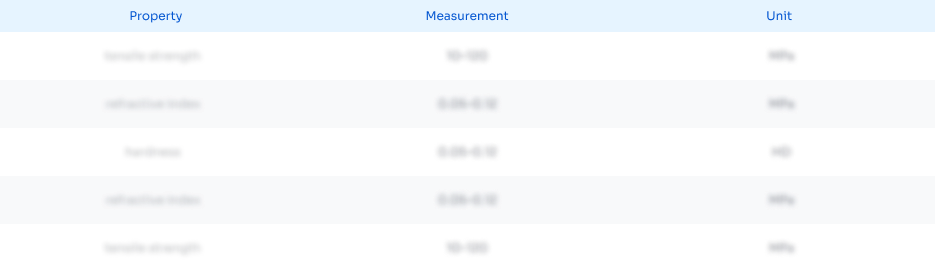
Abstract
Description
Claims
Application Information
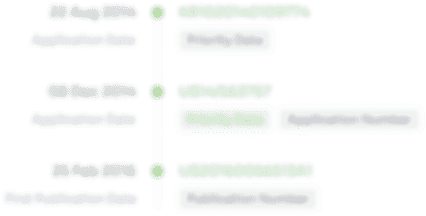
- Generate Ideas
- Intellectual Property
- Life Sciences
- Materials
- Tech Scout
- Unparalleled Data Quality
- Higher Quality Content
- 60% Fewer Hallucinations
Browse by: Latest US Patents, China's latest patents, Technical Efficacy Thesaurus, Application Domain, Technology Topic, Popular Technical Reports.
© 2025 PatSnap. All rights reserved.Legal|Privacy policy|Modern Slavery Act Transparency Statement|Sitemap|About US| Contact US: help@patsnap.com