Quantification method and system based on neural network difference
A technology of neural network and quantization method, which is applied in the field of quantization method and system based on neural network difference, which can solve the problems of performance loss, large number of deep neural network model parameters, and high precision requirements, so as to maintain performance and achieve extremely low bit compression Effect
- Summary
- Abstract
- Description
- Claims
- Application Information
AI Technical Summary
Problems solved by technology
Method used
Image
Examples
Embodiment 1
[0040] Such as figure 1 , image 3 As shown, the present invention provides a quantization method based on neural network difference, which specifically includes:
[0041] In the process of training the current network model, select the pre-trained network model and network structure of the relevant problem as the initialization of the current model;
[0042] In order to make full use of the pre-training model to reduce the size of the network model, the training parameter expression of the network model is used as the change amount based on the initialization model parameters;
[0043] Specifically, suppose the convolution parameters of the i-th layer of the pre-trained model are expressed as The convolution parameters of the newly trained model are denoted as W (i) . Under such assumptions, the operation of the current convolutional layer is expressed as:
[0044]
[0045] Among them, the network model L (i) Indicates the output of the i-th layer of the network mod...
Embodiment 2
[0063] The invention provides a quantization method based on neural network difference,
[0064] The network structure used is the SRCNN network model, where SRCNN is a three-layer convolutional neural network model. Specifically, the convolution kernel size of the first layer network model is 9×9, which is mainly used to extract the texture information on the input image; the convolution kernel size of the second layer network model is 1×1, which is mainly used to transform the input The characteristics of the image; the convolution kernel size of the last layer network model is 5×5, which is used to reconstruct the output image. After the first layer network model and the second layer network model, the ReLU activation function is added to increase the nonlinear transformation capability of the neural network.
[0065] The settings of the image quality restoration problem tested by the present invention are as follows. The images tested by the present invention are divided...
PUM
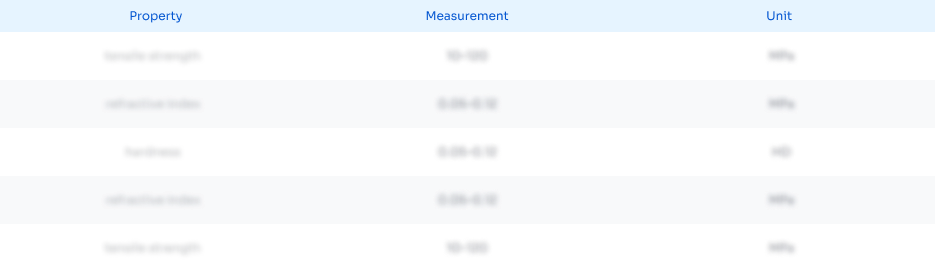
Abstract
Description
Claims
Application Information
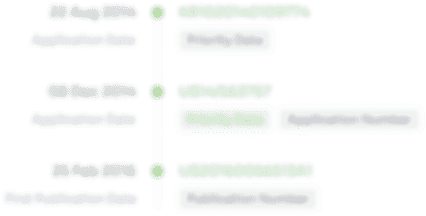
- R&D
- Intellectual Property
- Life Sciences
- Materials
- Tech Scout
- Unparalleled Data Quality
- Higher Quality Content
- 60% Fewer Hallucinations
Browse by: Latest US Patents, China's latest patents, Technical Efficacy Thesaurus, Application Domain, Technology Topic, Popular Technical Reports.
© 2025 PatSnap. All rights reserved.Legal|Privacy policy|Modern Slavery Act Transparency Statement|Sitemap|About US| Contact US: help@patsnap.com