Hot-rolled strip steel surface defect classification method based on convolutional neural network
A technology of convolutional neural network and hot-rolled steel strip, which is applied in the direction of biological neural network model, neural architecture, image analysis, etc., can solve the problems of memory occupation, low classification accuracy, and large system consumption, so as to reduce system consumption and Effects of memory usage, ease of gradient dispersion, and faster convergence
- Summary
- Abstract
- Description
- Claims
- Application Information
AI Technical Summary
Problems solved by technology
Method used
Image
Examples
Embodiment Construction
[0069] In order to further understand the content of the present invention, the present invention will be described in detail in conjunction with the accompanying drawings and embodiments.
[0070] The method for classifying surface defects of hot-rolled strip steel based on the convolutional neural network of the present invention takes the identification of surface defects of hot-rolled strip steel as an example, such as figure 1 As shown, the steps are as follows:
[0071] S1: Obtain typical image samples of hot-rolled strip surface defects from the NEU database, and preprocess the samples.
[0072] The NEU database refers to the (Northeastern University, Northeastern University) surface defect database, in which images of six typical surface defects on the hot-rolled strip surface can be obtained, such as figure 2 As shown, in the data image, there are 6 typical hot-rolled strip surface defect images including cracks, pressed scale, pockmarks, plaques, inclusions, and sc...
PUM
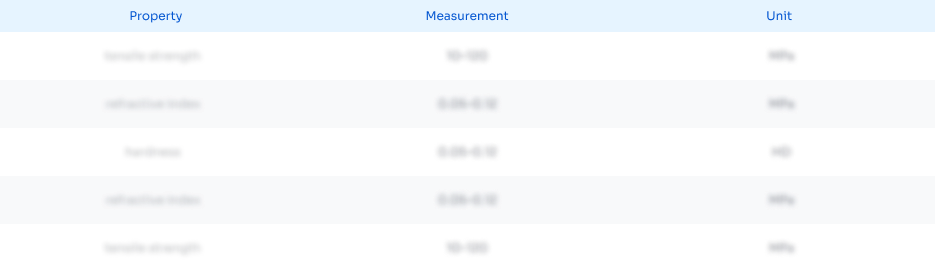
Abstract
Description
Claims
Application Information
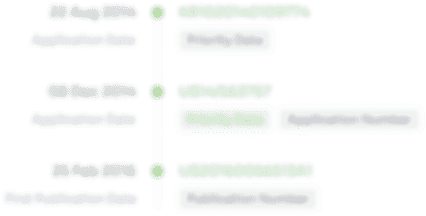
- R&D Engineer
- R&D Manager
- IP Professional
- Industry Leading Data Capabilities
- Powerful AI technology
- Patent DNA Extraction
Browse by: Latest US Patents, China's latest patents, Technical Efficacy Thesaurus, Application Domain, Technology Topic, Popular Technical Reports.
© 2024 PatSnap. All rights reserved.Legal|Privacy policy|Modern Slavery Act Transparency Statement|Sitemap|About US| Contact US: help@patsnap.com