Robust acoustic scene recognition method based on local learning
An acoustic scene and partial learning technology, applied in the field of acoustic scene recognition, can solve problems such as unbalanced number of samples in different channels, mismatched audio channels, and low accuracy of acoustic scene recognition, and solve the problem of unbalanced number of device categories and fast Computing speed, easy-to-implement effects
- Summary
- Abstract
- Description
- Claims
- Application Information
AI Technical Summary
Problems solved by technology
Method used
Image
Examples
specific Embodiment approach 1
[0017] Specific implementation manner 1: This implementation manner provides a robust acoustic scene recognition method based on local learning, which specifically includes the following steps:
[0018] Step 1. Collect sound signals of different acoustic scenes at a sampling frequency of 44.1KHz and perform frequency domain feature extraction. The collected audio is divided into frame sequences with a frame length of 40ms. The 40-dimensional FBank (filtered) is extracted from each frame of data. Set) feature to establish a training sample set;
[0019] Step 2: Preprocessing the feature data extracted in Step 1:
[0020] Calculate the mean and standard deviation in each dimension for the features extracted in step 1, as attached figure 1 As shown, calculate the mean value μ for all samples along the time axis, and calculate the standard deviation σ in the same way; use the obtained mean value and standard deviation to normalize all features;
[0021] Step 3: Channel adaptation and data...
specific Embodiment approach 2
[0025] Specific embodiment two: This embodiment is different from specific embodiment one in that all the features normalized by using the mean and standard deviation obtained in step two are specifically:
[0026] Use the obtained mean and standard deviation to normalize the characteristic data according to the following formula:
[0027]
[0028] Where x norm Indicates the normalized data, μ is the mean, σ is the standard deviation; x is the characteristic data.
[0029] The other steps and parameters are the same as in the first embodiment.
specific Embodiment approach 3
[0030] Specific embodiment three: This embodiment is different from specific embodiment two in that the mean value shift in step three is specifically:
[0031] Add the difference ε to the normalized data with probability p:
[0032]
[0033] Among them, μ most Represents the data mean vector of the device with the largest number of samples; N represents the number of devices other than the device with the largest number of samples, μ i Represents the data mean vector of the i-th device except for the device with the largest number of samples; i=1,...,N; in order to increase the robustness of the system, not all data are added by difference, but by probability p plus, p∈[0,1].
[0034] The other steps and parameters are the same as in the second embodiment.
PUM
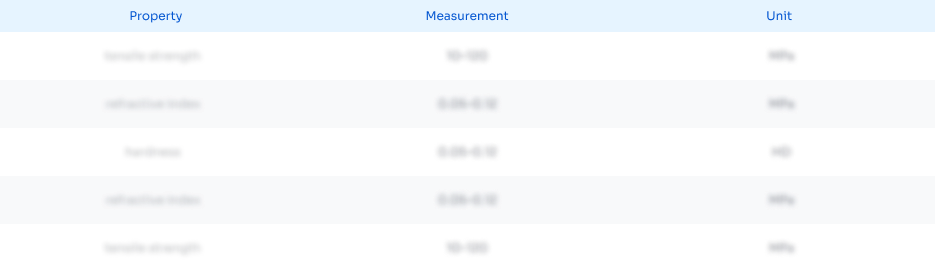
Abstract
Description
Claims
Application Information
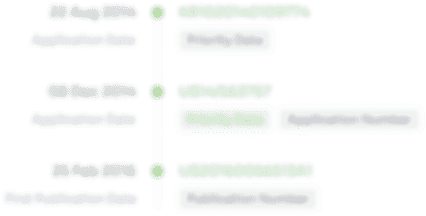
- R&D Engineer
- R&D Manager
- IP Professional
- Industry Leading Data Capabilities
- Powerful AI technology
- Patent DNA Extraction
Browse by: Latest US Patents, China's latest patents, Technical Efficacy Thesaurus, Application Domain, Technology Topic, Popular Technical Reports.
© 2024 PatSnap. All rights reserved.Legal|Privacy policy|Modern Slavery Act Transparency Statement|Sitemap|About US| Contact US: help@patsnap.com