Large-scene airborne point cloud semantic modeling method
A modeling method and technology for large scenes, applied in 3D modeling, image data processing, instruments, etc., can solve the problems of weakening the salience of the model boundary, seldom considering the global structural expression of the building, sacrificing the geometric accuracy of the model, etc.
- Summary
- Abstract
- Description
- Claims
- Application Information
AI Technical Summary
Problems solved by technology
Method used
Image
Examples
Embodiment Construction
[0106] The technical solution of the present invention will be further described below in conjunction with the accompanying drawings.
[0107] Such as figure 1 The overall framework of the airborne point cloud semantic modeling method for large scenes is composed of four parts: ALS point cloud scene classification, architectural semantic identification, architectural semantic reconstruction and accuracy evaluation.
[0108] (1) ALS point cloud scene classification:
[0109] In order to realize the classification of ALS point clouds, the present invention establishes a three-dimensional capsule network deep learning model based on multi-level objects, and obtains a multi-level classification framework of ALS point clouds. With the help of the capsule network model with more robust performance in deep learning theory, the framework makes full use of the advantages of deep learning in salient feature expression, and designs a deep learning feature expression method based on mult...
PUM
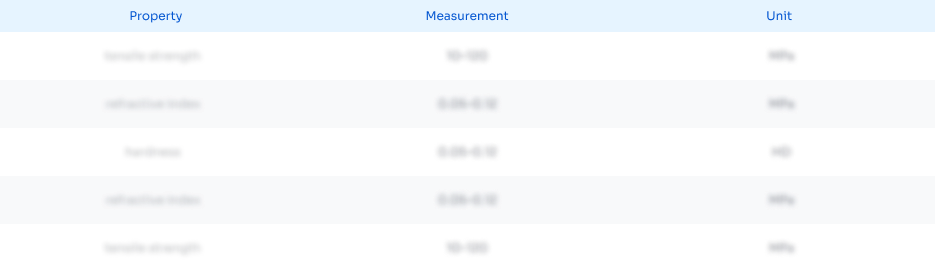
Abstract
Description
Claims
Application Information
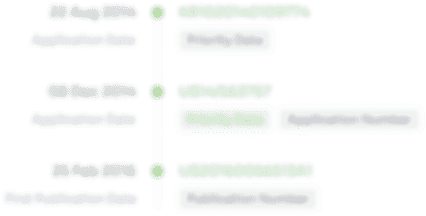
- R&D Engineer
- R&D Manager
- IP Professional
- Industry Leading Data Capabilities
- Powerful AI technology
- Patent DNA Extraction
Browse by: Latest US Patents, China's latest patents, Technical Efficacy Thesaurus, Application Domain, Technology Topic, Popular Technical Reports.
© 2024 PatSnap. All rights reserved.Legal|Privacy policy|Modern Slavery Act Transparency Statement|Sitemap|About US| Contact US: help@patsnap.com