Methods for treating cancer and predicting drug responsiveness in cancer patients
A responsive, cancer-based technology with applications in biochemical devices and methods, drug combinations, drug delivery, etc.
- Summary
- Abstract
- Description
- Claims
- Application Information
AI Technical Summary
Problems solved by technology
Method used
Image
Examples
Embodiment 1
[0217] Example 1. Identification of biomarkers of sensitivity and resistance to cisplatin using the Affymetrix HG-U133A array.
[0218] A key component of LiPlaCis is cisplatin, a common cancer drug encapsulated in a liposomal formulation. Obviously if cisplatin doesn't work, then LiPlaCis won't work on the tumor. Therefore, it is possible to predict a portion of the response to LiPlaCis as the response to cisplatin. Liposome delivery to tumor cells is a separate part of the requirement for LiPlaCis to function and can be modeled separately, for example, by measuring sPLA2 on the surface of tumor cells.
[0219] DNA microarray measurements of 60 cancer cell lines from the NCI60 dataset were performed using the Affymetrix HG-U133A array and normalized logit. Each array was logit transformed followed by Z transformation to mean zero and SD 1 and correlated with growth inhibition (log(GI50)). LiPlaCis growth inhibition data against the same cell lines were downloaded from the ...
Embodiment 2
[0220] Example 2. Identification of biomarkers sensitive and resistant to LiPlaCis using the Affymetrix HG-U133A array.
[0221] DNA microarray measurements of 60 cancer cell lines of the NCI60 dataset were also performed using the HG-U133_Plus_2 array and normalized logit. Each array was logit transformed followed by Z transformation to mean zero and SD 1 and correlated with growth inhibition (log(GI50)). LiPlaCis growth inhibition data against the same cell lines were downloaded from the National Cancer Institute. The expression of each gene in each cell line correlated with the growth (log(GI50)) of those cell lines in the presence of LiPlaCis. Covariance (Pearson's correlation coefficient multiplied by standard deviation) was then determined to identify genes positively and negatively associated with sensitivity to LiPlaCis. Tables 4 and 5 show the highest positive correlation genes (biomarkers with sensitivity) and negative correlation genes (biomarkers with resistance)...
Embodiment 3
[0222] Example 3. Predicting responsiveness to LiPlaCis in various cancer patient populations.
[0223] The mRNA-based predictors responsive to LiPlaCis developed according to the method of the present invention were applied to 3,522 patients with various cancers. Pre-treatment measurements of gene expression were performed with Affymetrix arrays for each patient. The predicted LiPlaCis sensitivity for each patient was calculated as the difference between the average expression level of sensitive biomarkers (Table 2) and resistant biomarkers (Table 3) in the patients. When patients were grouped by cancer type, cancer types predicted to be more responsive to LiPlaCis were identified ( figure 1 ).
[0224] Across 27 different cancer types, solid tumor cancers were predicted to be more responsive to LiPlaCis therapy than hematologic cancers. Specifically, patients with hematological cancer types were predicted to respond to LiPlaCis treatment.
[0225] figure 1The median val...
PUM
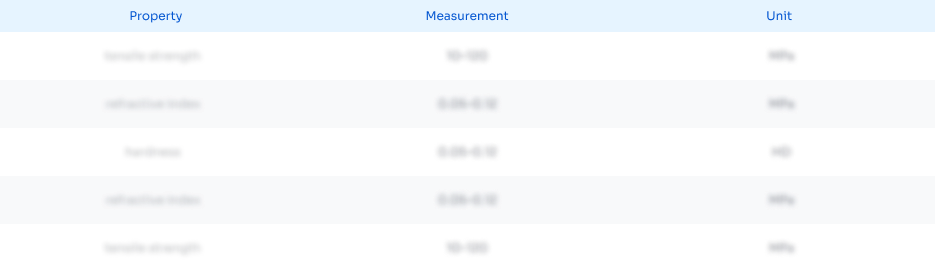
Abstract
Description
Claims
Application Information
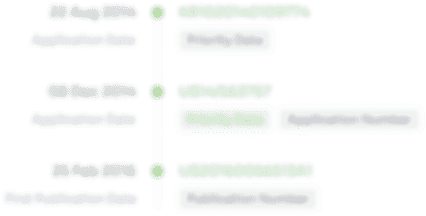
- R&D Engineer
- R&D Manager
- IP Professional
- Industry Leading Data Capabilities
- Powerful AI technology
- Patent DNA Extraction
Browse by: Latest US Patents, China's latest patents, Technical Efficacy Thesaurus, Application Domain, Technology Topic, Popular Technical Reports.
© 2024 PatSnap. All rights reserved.Legal|Privacy policy|Modern Slavery Act Transparency Statement|Sitemap|About US| Contact US: help@patsnap.com