A convolutional echo state network time sequence classification method based on a multi-head self-attention mechanism
A technology of echo state network and classification method, applied in the field of reserve pool computing and neural network research, can solve problems such as affecting model performance, inability to obtain performance, etc., to achieve the effect of reservation-free training
- Summary
- Abstract
- Description
- Claims
- Application Information
AI Technical Summary
Problems solved by technology
Method used
Image
Examples
Embodiment
[0052] Such as figure 1 As shown, this embodiment discloses a multi-variable time-series classification method based on the multi-head self-attention mechanism of the convolutional echo state network. This method introduces a multi-head self-attention mapping mechanism into the traditional echo state network, performs multiple high-dimensional projections on the input time series and integrates high-dimensional feature global space-time encoding, and realizes the capture of complex time series features. Finally, a shallow layer The convolutional neural network achieves high-precision classification. The convolutional echo state network model based on the multi-head self-attention mechanism is a new type of reserve pool calculation model applied to time series classification. The model establishment process is as follows: figure 2 shown, including the following steps:
[0053] S1. Network initialization, determine the number of reserve pools, and initialize the internal para...
PUM
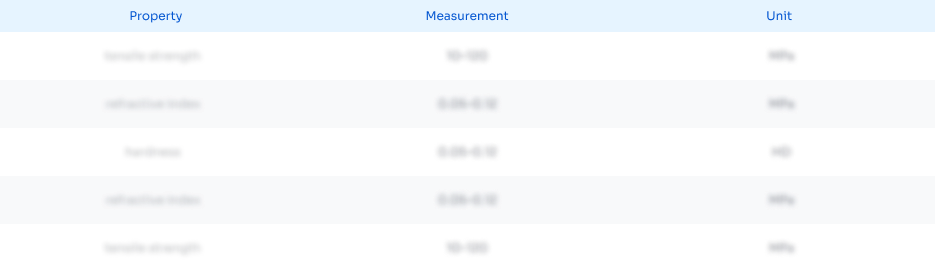
Abstract
Description
Claims
Application Information
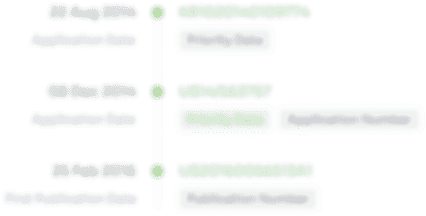
- R&D Engineer
- R&D Manager
- IP Professional
- Industry Leading Data Capabilities
- Powerful AI technology
- Patent DNA Extraction
Browse by: Latest US Patents, China's latest patents, Technical Efficacy Thesaurus, Application Domain, Technology Topic, Popular Technical Reports.
© 2024 PatSnap. All rights reserved.Legal|Privacy policy|Modern Slavery Act Transparency Statement|Sitemap|About US| Contact US: help@patsnap.com