A high-voltage wire defect detection method based on a regional convolutional neural network
A convolutional neural network and high-voltage wire technology, which is applied in the field of high-voltage wire defect detection of convolutional neural network, can solve the problems of low efficiency and accuracy of wire defect recognition, difficulty in making full use of inspection images, etc.
- Summary
- Abstract
- Description
- Claims
- Application Information
AI Technical Summary
Problems solved by technology
Method used
Image
Examples
Embodiment Construction
[0048] Since the references of the region-based convolutional neural network algorithm are almost all in English, in order to avoid ambiguity caused by translation, Chinese and English abbreviations are hereby compared.
[0049] The present invention will be further described below in conjunction with the accompanying drawings and embodiments.
[0050] In the present invention, the training of the network and the detection of test samples are all based on the open source Tensorflow SLIM library. Tensorflow is a clear and efficient deep learning framework with excellent readability, simplicity and performance, and directly integrates the convolutional network neural layer. Due to the characteristics of the deep convolutional network itself, using GPU to accelerate the operation can greatly shorten the algorithm training time. Tensorflow also provides corresponding interfaces. The complete training process will combine figure 2 Be specific.
[0051] In the embodiment of the...
PUM
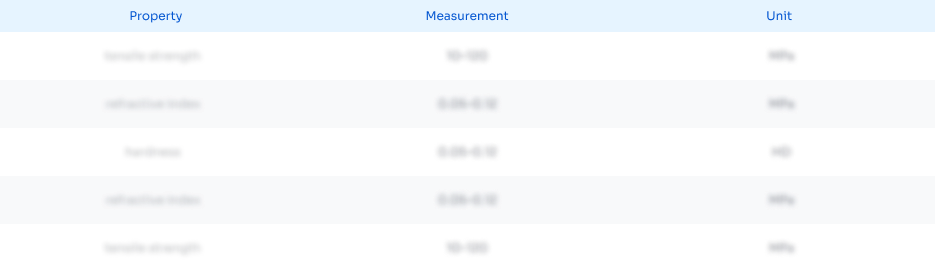
Abstract
Description
Claims
Application Information
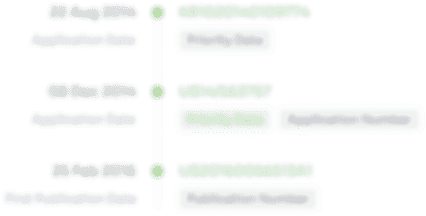
- R&D Engineer
- R&D Manager
- IP Professional
- Industry Leading Data Capabilities
- Powerful AI technology
- Patent DNA Extraction
Browse by: Latest US Patents, China's latest patents, Technical Efficacy Thesaurus, Application Domain, Technology Topic, Popular Technical Reports.
© 2024 PatSnap. All rights reserved.Legal|Privacy policy|Modern Slavery Act Transparency Statement|Sitemap|About US| Contact US: help@patsnap.com