Failure prediction method of roller bearing based on partial least squares extreme learning machine
A partial least squares, extreme learning machine technology, applied in the field of rolling bearing fault prediction based on partial least squares extreme learning machine, can solve problems such as time-consuming and labor-intensive, difficult to find the type of regression equation, and achieve a small root mean square error , to solve the problem of modal aliasing, the effect of suppressing noise
- Summary
- Abstract
- Description
- Claims
- Application Information
AI Technical Summary
Problems solved by technology
Method used
Image
Examples
specific Embodiment approach 1
[0032] Specific implementation mode one: as figure 1 As shown, the rolling bearing fault prediction method based on the partial least squares extreme learning machine in this embodiment, the method includes the following steps:
[0033] Step 1: Signal noise reduction based on half normal distribution and EWT;
[0034] Analyze the limitations of the traditional EWT for signal noise reduction, and propose a new half-normal distribution (the random variables affected by a large number of independent uniform small effects all obey the half-normal distribution. Because of this, in the field of mechanical failure, mechanical vibration is used Treated as a half-normal distribution.) A theoretical algorithm combined with EWT;
[0035] Step 2: Dimensionality reduction of rolling bearing data based on C-ISOMAP (establishing a fuzzy C clustering model, using the popular ISOMAP algorithm to reduce the dimensionality of nonlinear data, and the processed low-dimensional data can maintain t...
specific Embodiment approach 2
[0039] Specific implementation mode 2: This implementation mode is a further description of specific implementation mode 1;
[0040] The specific steps of step 1 (signal denoising based on EWT and half-normal distribution) are as follows:
[0041] Step 11: Set the sampling time and frequency for the acceleration sensor installed on the rolling bearing seat. Then determine the number of sensor channels, and collect vibration signals of rolling bearings under different damage states. Then the fault signal obtained by preprocessing is used as the input signal of fault prediction.
[0042] Step 1 and 2: For the problem of ambiguous positioning of the EWT interval, there are many AM-FM components after division, and there is a lack of optimization process. It is necessary to establish a screening index to select the optimal component. Here, the semi-normal distribution is used for optimization. The expression as follows:
[0043]
[0044] In the formula, σ represents the stan...
specific Embodiment approach 3
[0046] Specific implementation mode three: this implementation mode is a further description of specific implementation mode one;
[0047] The specific steps of step 2 (dimensional reduction of rolling bearing data based on C-ISOMAP) are as follows:
[0048] Step 21: During the entire monitoring process of the rolling bearing, not only the different damage degrees of the bearing can be distinguished, but also the degradation trend of the rolling bearing can be predicted and classified. The normal vibration signal and the final failure signal of the rolling bearing are used as training data to establish a fuzzy C clustering model.
[0049] Step 22: Fault feature extraction: Extract the dimensioned and dimensionless feature values of the initial damage stage, moderate damage stage, and severe damage stage of the denoised data to form a high-dimensional feature set representing the fault signal.
[0050] Step 2 and 3: Take the intrinsic popular feature: Since the topological s...
PUM
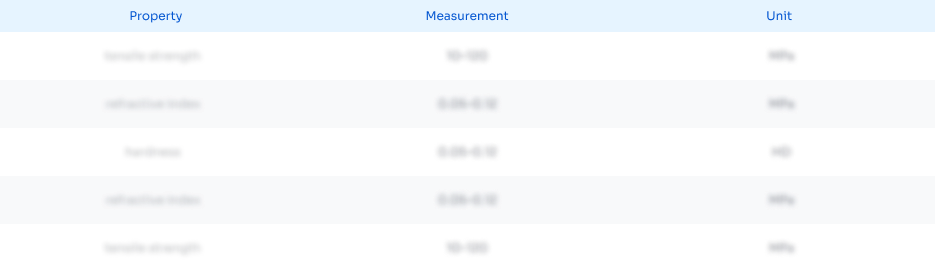
Abstract
Description
Claims
Application Information
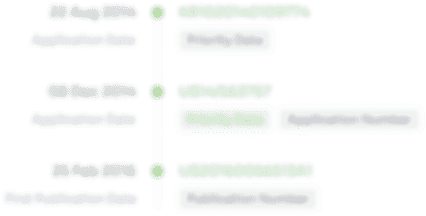
- R&D Engineer
- R&D Manager
- IP Professional
- Industry Leading Data Capabilities
- Powerful AI technology
- Patent DNA Extraction
Browse by: Latest US Patents, China's latest patents, Technical Efficacy Thesaurus, Application Domain, Technology Topic, Popular Technical Reports.
© 2024 PatSnap. All rights reserved.Legal|Privacy policy|Modern Slavery Act Transparency Statement|Sitemap|About US| Contact US: help@patsnap.com