Small sample image recognition method based on deep learning
A sample image, image recognition technology, applied in the field of image recognition
- Summary
- Abstract
- Description
- Claims
- Application Information
AI Technical Summary
Problems solved by technology
Method used
Image
Examples
Embodiment Construction
[0057] Such as figure 1 , 2 As shown, the method for small sample image recognition based on deep learning of the present invention includes the following steps: 1. Divide the training set; 2. Generate noisy images; 3. Pre-train the prototype space discrimination network; 4. Train the deception image generation network; 5. , Training the prototype space discriminant network; 6. Repeat steps 4 and 5 for cross iteration training until the preset number of iterations is reached or the accuracy is no longer improved; 7. Image category recognition.
[0058] One, divide the training set
[0059] The sample images in the training set are randomly divided into support set S and query set Q. The number of sample images in each category in the training set is generally not less than 600; among them, the support set Indicates that the support set S contains sample images of n categories, where To support the collection of sample images belonging to category k in the set S, Is the mth sampl...
PUM
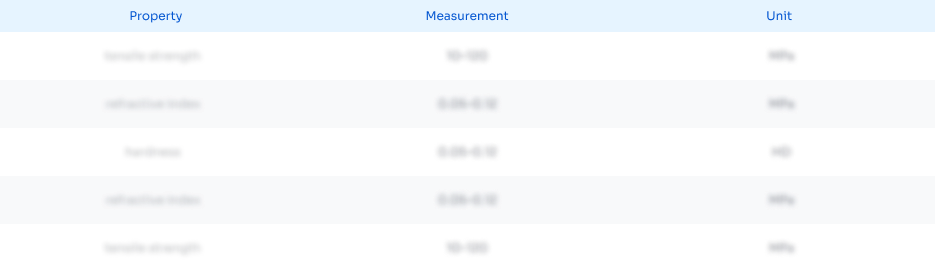
Abstract
Description
Claims
Application Information
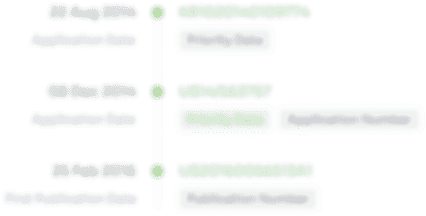
- R&D Engineer
- R&D Manager
- IP Professional
- Industry Leading Data Capabilities
- Powerful AI technology
- Patent DNA Extraction
Browse by: Latest US Patents, China's latest patents, Technical Efficacy Thesaurus, Application Domain, Technology Topic, Popular Technical Reports.
© 2024 PatSnap. All rights reserved.Legal|Privacy policy|Modern Slavery Act Transparency Statement|Sitemap|About US| Contact US: help@patsnap.com