Privacy protection method and system based on power system edge computing
An edge computing and privacy protection technology, applied in the direction of digital data protection, complex mathematical operations, platform integrity maintenance, etc., can solve real-time sensitive data attacks, small storage space and other problems, to reduce bandwidth and data travel time to and from the cloud server , the effect of reducing the risk of leakage
- Summary
- Abstract
- Description
- Claims
- Application Information
AI Technical Summary
Problems solved by technology
Method used
Image
Examples
Embodiment 1
[0027] First of all, in the power system, users are divided into important users and ordinary users. The data generated by the power terminals of ordinary users does not need to be protected for privacy. It is only necessary to protect the privacy of the data generated by the power terminals of important users. The data generated by the power terminal is divided into sensitive data and non-sensitive data. Since sensitive data occupies a small part of the entire data set and non-sensitive data accounts for the majority, the present invention uses an improved algorithm based on non-negative matrix decomposition to perform data disturbance on sensitive data. In this way, the privacy protection of sensitive data can be achieved.
[0028] Such as figure 2 As shown, a privacy protection system based on power system edge computing includes power terminals, edge computing devices, and cloud servers. The edge computing devices are respectively connected to the cloud server and the po...
Embodiment 2
[0030] The specific steps of the present invention to disturb the data are as follows:
[0031] Step 1: Important data in the original data set D=[d 1 , d 2 ,...,d t ,...,d n ] contains sensitive attributes and non-sensitive attributes, first set the value of non-sensitive data to 0, and the sensitive data remains unchanged, assuming d 1 , d 2 and d n For non-sensitive data, get a new matrix D1=[0,0,…,d t ,...,0].
[0032] d t is a vector of m×1;
[0033] Step 2: Perform non-negative matrix decomposition on D1 to obtain matrix W m×kand H k×n (kk ,D k ≈D1.
[0034] Step 4: Add the original non-sensitive data to the sensitive disturbance matrix D' to obtain the final disturbance data D2.
Embodiment 3
[0036] On the basis of embodiment 2, the present invention adds step 3 again after step 2, and all the other steps are unchanged, specifically as follows:
[0037] Step 3. Only the data disturbance generated by the non-negative matrix decomposition in step 2 cannot achieve a better privacy protection effect. In this embodiment, random disturbance is performed on W in step 2 to obtain W', and W' is multiplied by H to obtain D '.
[0038] The privacy protection effect of this embodiment is better than that of Embodiment 2, but the computational complexity is slightly higher than that of Embodiment 2.
[0039] As an optional mode, the improved algorithm of the present invention based on non-negative matrix factorization is as follows:
[0040] Input the original sample matrix D, the rows of D represent samples, the columns represent attributes, and the elements in D are all non-negative. The parameter is k.
[0041] Output perturbation sample matrix D2
[0042] Classify the ...
PUM
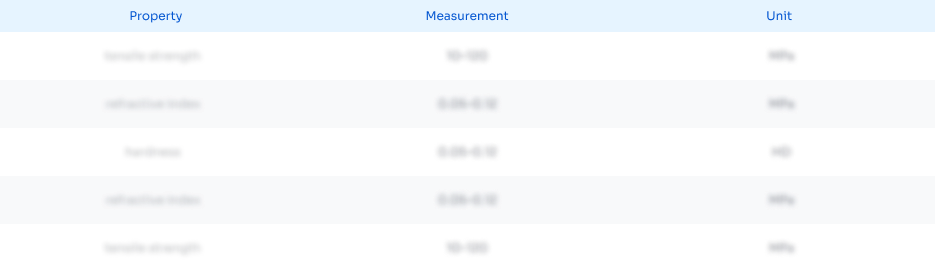
Abstract
Description
Claims
Application Information
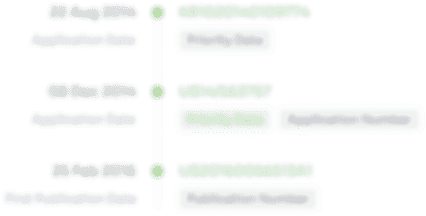
- R&D Engineer
- R&D Manager
- IP Professional
- Industry Leading Data Capabilities
- Powerful AI technology
- Patent DNA Extraction
Browse by: Latest US Patents, China's latest patents, Technical Efficacy Thesaurus, Application Domain, Technology Topic, Popular Technical Reports.
© 2024 PatSnap. All rights reserved.Legal|Privacy policy|Modern Slavery Act Transparency Statement|Sitemap|About US| Contact US: help@patsnap.com