Fine crack segmentation method based on generative adversarial networks
A network and crack technology, applied in biological neural network models, image analysis, image data processing, etc., can solve the problems that feature detection cannot be guaranteed effectively, training and testing take a lot of time, and achieve the effect of high graphics quality.
- Summary
- Abstract
- Description
- Claims
- Application Information
AI Technical Summary
Problems solved by technology
Method used
Image
Examples
Embodiment Construction
[0045] In order to further illustrate the technical means and effects adopted by the present invention to achieve the intended purpose, the specific implementation, structural features and effects of the present invention will be described in detail below in conjunction with the accompanying drawings and examples.
[0046] The generative confrontation network consists of two parts, the generator and the discriminator. The discriminator is a simple convolutional neural network model, which takes real images and fake images constructed by the generator as input, and extracts features from the input data through a series of convolutional layers, excitation layers, normalization layers, and pooling layers , and finally output the probability value of the [0, 1] interval; the generator is a reverse convolutional neural network model, through a series of deconvolution layers for upsampling, combined with the excitation layer, the low-dimensional vector is converted into a real Vecto...
PUM
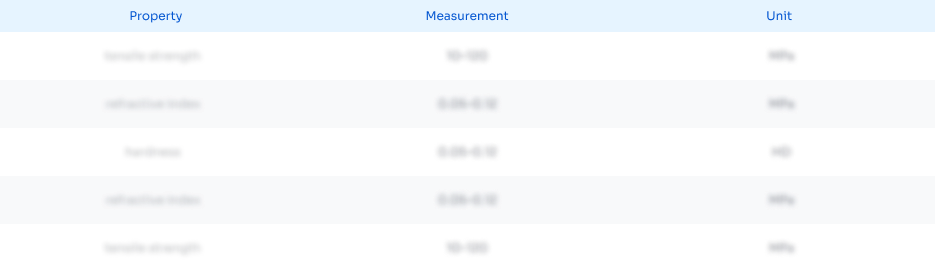
Abstract
Description
Claims
Application Information
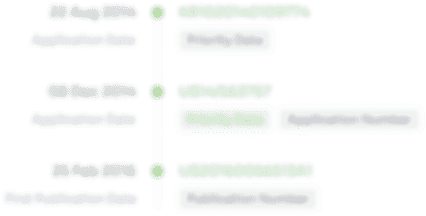
- R&D Engineer
- R&D Manager
- IP Professional
- Industry Leading Data Capabilities
- Powerful AI technology
- Patent DNA Extraction
Browse by: Latest US Patents, China's latest patents, Technical Efficacy Thesaurus, Application Domain, Technology Topic, Popular Technical Reports.
© 2024 PatSnap. All rights reserved.Legal|Privacy policy|Modern Slavery Act Transparency Statement|Sitemap|About US| Contact US: help@patsnap.com