Semantic segmentation of road scene based on multi-scale perforated convolutional neural network
A convolutional neural network and semantic segmentation technology, applied in the field of semantic segmentation of road scenes based on multi-scale atrous convolutional neural network, can solve problems such as low segmentation accuracy, reduced image feature information, and rough restored edge information
- Summary
- Abstract
- Description
- Claims
- Application Information
AI Technical Summary
Problems solved by technology
Method used
Image
Examples
Embodiment Construction
[0052] The present invention will be further described in detail below in conjunction with the accompanying drawings and embodiments.
[0053] A road scene semantic segmentation method based on a multi-scale atrous convolutional neural network proposed by the present invention, its overall realization block diagram is as follows figure 1 As shown, it includes two processes of training phase and testing phase;
[0054] The specific steps of the described training phase process are:
[0055] Step 1_1: Select Q original road scene images and the real semantic segmentation images corresponding to each original road scene image, and form a training set, and record the qth original road scene image in the training set as {I q (i,j)}, combine the training set with {I q (i, j)} corresponding to the real semantic segmentation image is denoted as Then, the existing one-hot encoding technology (one-hot) is used to process the real semantic segmentation images corresponding to each or...
PUM
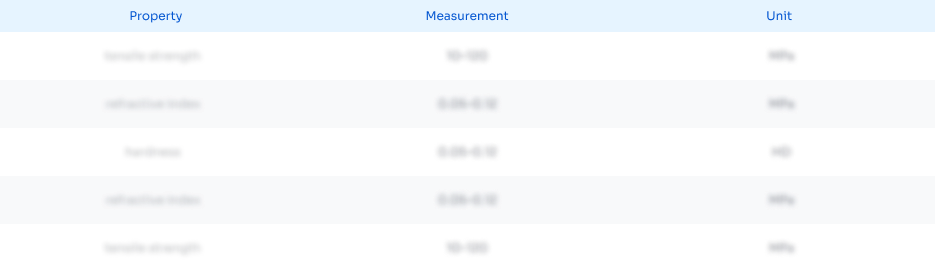
Abstract
Description
Claims
Application Information
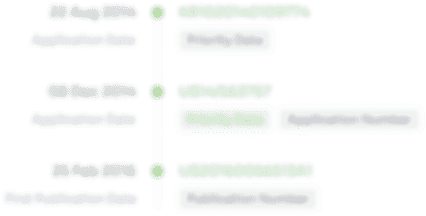
- R&D Engineer
- R&D Manager
- IP Professional
- Industry Leading Data Capabilities
- Powerful AI technology
- Patent DNA Extraction
Browse by: Latest US Patents, China's latest patents, Technical Efficacy Thesaurus, Application Domain, Technology Topic, Popular Technical Reports.
© 2024 PatSnap. All rights reserved.Legal|Privacy policy|Modern Slavery Act Transparency Statement|Sitemap|About US| Contact US: help@patsnap.com